As 2024 comes to an end, the momentum behind the developments of Generative AI shows no signs of slowing down. The adoption of AI remains at the top of enterprise priorities, with leaders striving to streamline workflows, enhance employee productivity, and unlock new efficiencies across their organizations. Over the past year, the majority of businesses we talked to have established dedicated teams for GenAI and have discovered many use cases. However, in many cases, inherent security risks prevented GenAI apps from being launched into production. Security teams were still in the early stages of learning how to effectively secure these AI systems, grappling with challenges like sensitive data leakage, prompt injections, and unintended outputs that can harm brand reputation. By learning the nuances of LLM behavior and with a growing ecosystem of proprietary and open-source tools designed to address AI security and safety risks, AI practitioners are now better equipped than ever to build secure and reliable AI systems as we move into the new year.
AI Security: From General to Vertical Solutions
The current AI security landscape remains dominated by general-purpose solutions, with very few providers focusing deeply on specific industries like fintech, healthcare, legal, or automotive. This broad approach has been effective so far, as public exploits targeting specific industries remain rare. Additionally, vertical-specific LLMs have yet to gain significant traction, partly due to their relatively modest performance on domain-specific benchmarks, which has delayed the push for deeper specialization.
However, this is set to change in 2025. The increasing complexity of domain-specific agentic AI workflows and specialized LLMs are driving demand for more vertical security solutions. Stakeholders in every industry have identified specific risks that are top security priorities, making general-purpose solutions less viable. In healthcare, for example, LLMs could generate inaccurate clinical recommendations, potentially harming patients and exposing providers to legal liabilities. In finance, manipulated AI systems might misclassify fraudulent activities, enabling unauthorized transactions or money laundering. To address these unique challenges, AI security providers must focus on delivering tailored protections, driving the industry toward more specialized solutions.
As we look ahead to the developments in 2025, let’s explore the trends that will not only reshape how enterprises leverage AI but also redefine the course of the AI security industry.
As 2024 comes to an end, the momentum behind the developments of Generative AI shows no signs of slowing down. The adoption of AI remains at the top of enterprise priorities, with leaders striving to streamline workflows, enhance employee productivity, and unlock new efficiencies across their organizations. Over the past year, the majority of businesses we talked to have established dedicated teams for GenAI and have discovered many use cases. However, in many cases, inherent security risks prevented GenAI apps from being launched into production. Security teams were still in the early stages of learning how to effectively secure these AI systems, grappling with challenges like sensitive data leakage, prompt injections, and unintended outputs that can harm brand reputation. By learning the nuances of LLM behavior and with a growing ecosystem of proprietary and open-source tools designed to address AI security and safety risks, AI practitioners are now better equipped than ever to build secure and reliable AI systems as we move into the new year.
AI Security: From General to Vertical Solutions
The current AI security landscape remains dominated by general-purpose solutions, with very few providers focusing deeply on specific industries like fintech, healthcare, legal, or automotive. This broad approach has been effective so far, as public exploits targeting specific industries remain rare. Additionally, vertical-specific LLMs have yet to gain significant traction, partly due to their relatively modest performance on domain-specific benchmarks, which has delayed the push for deeper specialization.
However, this is set to change in 2025. The increasing complexity of domain-specific agentic AI workflows and specialized LLMs are driving demand for more vertical security solutions. Stakeholders in every industry have identified specific risks that are top security priorities, making general-purpose solutions less viable. In healthcare, for example, LLMs could generate inaccurate clinical recommendations, potentially harming patients and exposing providers to legal liabilities. In finance, manipulated AI systems might misclassify fraudulent activities, enabling unauthorized transactions or money laundering. To address these unique challenges, AI security providers must focus on delivering tailored protections, driving the industry toward more specialized solutions.
As we look ahead to the developments in 2025, let’s explore the trends that will not only reshape how enterprises leverage AI but also redefine the course of the AI security industry.
As 2024 comes to an end, the momentum behind the developments of Generative AI shows no signs of slowing down. The adoption of AI remains at the top of enterprise priorities, with leaders striving to streamline workflows, enhance employee productivity, and unlock new efficiencies across their organizations. Over the past year, the majority of businesses we talked to have established dedicated teams for GenAI and have discovered many use cases. However, in many cases, inherent security risks prevented GenAI apps from being launched into production. Security teams were still in the early stages of learning how to effectively secure these AI systems, grappling with challenges like sensitive data leakage, prompt injections, and unintended outputs that can harm brand reputation. By learning the nuances of LLM behavior and with a growing ecosystem of proprietary and open-source tools designed to address AI security and safety risks, AI practitioners are now better equipped than ever to build secure and reliable AI systems as we move into the new year.
AI Security: From General to Vertical Solutions
The current AI security landscape remains dominated by general-purpose solutions, with very few providers focusing deeply on specific industries like fintech, healthcare, legal, or automotive. This broad approach has been effective so far, as public exploits targeting specific industries remain rare. Additionally, vertical-specific LLMs have yet to gain significant traction, partly due to their relatively modest performance on domain-specific benchmarks, which has delayed the push for deeper specialization.
However, this is set to change in 2025. The increasing complexity of domain-specific agentic AI workflows and specialized LLMs are driving demand for more vertical security solutions. Stakeholders in every industry have identified specific risks that are top security priorities, making general-purpose solutions less viable. In healthcare, for example, LLMs could generate inaccurate clinical recommendations, potentially harming patients and exposing providers to legal liabilities. In finance, manipulated AI systems might misclassify fraudulent activities, enabling unauthorized transactions or money laundering. To address these unique challenges, AI security providers must focus on delivering tailored protections, driving the industry toward more specialized solutions.
As we look ahead to the developments in 2025, let’s explore the trends that will not only reshape how enterprises leverage AI but also redefine the course of the AI security industry.
1. Agentic AI Workflows
In 2024, the majority of AI assistants developed across industries were retrieval-augmented generation (RAG) systems connected to databases, designed to support humans in completing specific tasks. These systems relied on standard LLM-based applications with limited autonomy and minimal internal functionality. However, 2025 is poised to bring a significant shift with the rise of Agentic AI systems. These systems are designed to autonomously perform complex, multi-step tasks on behalf of humans, leveraging advanced reasoning and internal functions to operate with minimal or no direct supervision. This evolution unlocks unprecedented possibilities for efficiency while introducing new risks and challenges for enterprises.
Different Types of Agentic AI Workflows
Autonomous Systems Without Human-in-the-Loop
These systems operate independently, making decisions and executing tasks without requiring human intervention.
Example Use Case: A logistics AI that autonomously manages supply chain operations, from inventory optimization to delivery scheduling.
Key Threat: Attackers could exploit task overload vulnerabilities by crafting tasks designed to overwhelm autonomous systems. This could lead to denial-of-service (DoS) scenarios, where the workflow iterates to its maximum allowable limits before completing an action. These exploits are particularly challenging to detect when the task appears to fulfill its intended purpose.
Collaborative Multi-Agent Systems
These workflows involve multiple agents working together, each specialized in different functions or roles, to achieve a shared objective.
Example Use Case: A customer service setup where one agent handles inquiries, another resolves technical issues, and a third processes payments.
Key Threat: In collaborative multi-agent systems, malicious actors might target the weakest link, exploiting a single vulnerable agent to disrupt the entire system. This type of systemic disruption could result in incomplete tasks or widespread operational failures, undermining the efficiency of the workflow.
Self-Optimizing Systems
These systems refine their processes over time, learning from feedback to improve their efficiency and outcomes.
Example Use Case: A personalized marketing AI that optimizes customer targeting and messaging strategies based on user engagement data.
Key Threat: Harmful optimization manipulation is another significant risk, where attackers provide deceptive feedback to misguide self-optimizing systems. By falsely signaling a preference for incorrect or harmful outputs, they can push the AI to adapt and optimize its behavior toward undesirable or damaging outcomes.
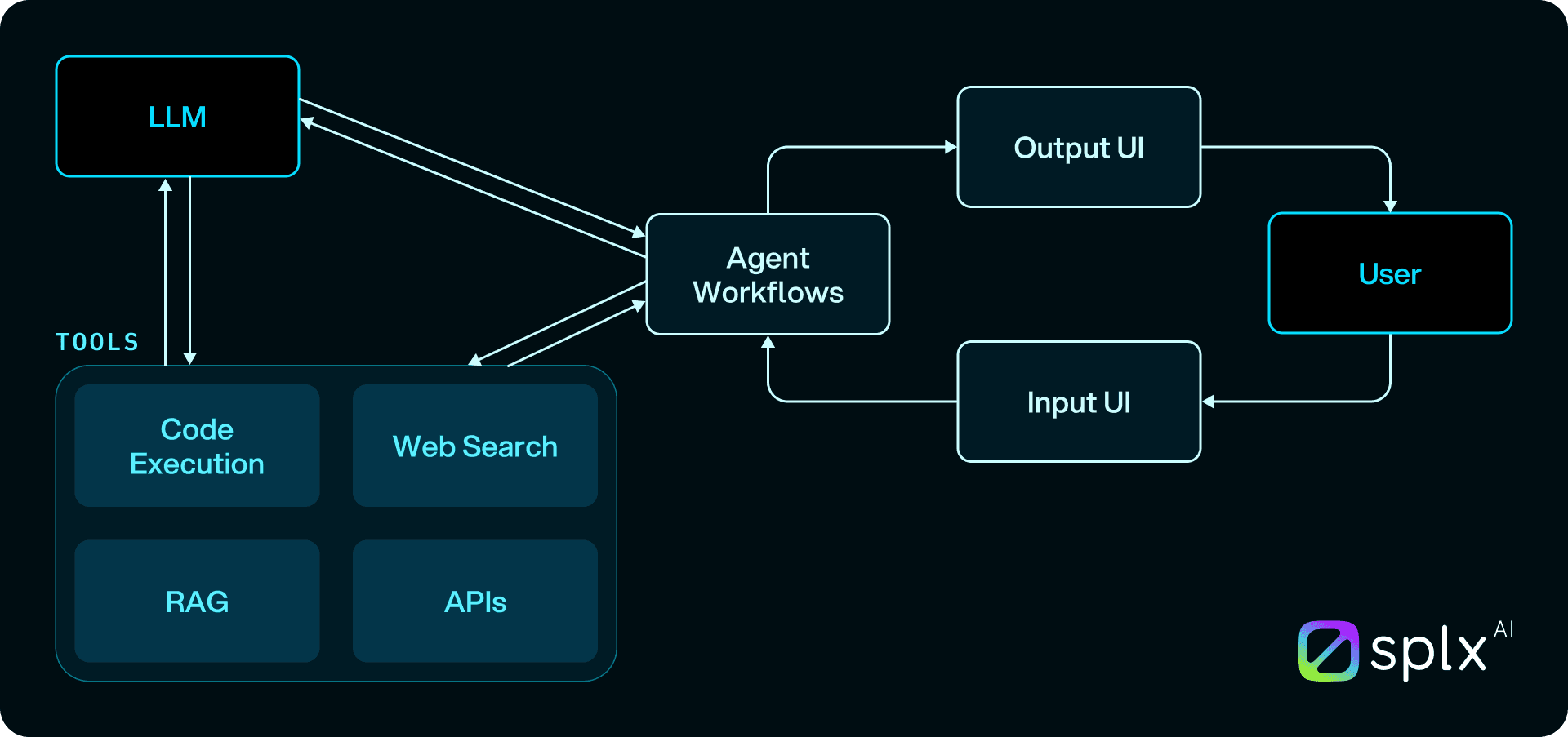
Why This Matters
The adoption of Agentic AI workflows represents a leap forward in operational efficiency:
Enhanced Efficiency: By autonomously executing tasks and refining actions based on real-time feedback, organizations can significantly reduce manual oversight, enabling faster decision-making and improved resource allocation.
New Attack Vectors: The increased autonomy introduces risks, as these systems may inadvertently bypass security policies or become entry points for sophisticated cyberattacks. Additionally, their complexity makes vulnerabilities harder to detect and mitigate.
Implications for AI Security
The rise of agentic AI workflows demands a rethinking of AI security strategies. These are some aspects enterprise security teams should prioritize:
Advanced Guardrails: Fine-tuned safeguards and hardened system prompts to ensure the AI’s behavior remains aligned with organizational policies and within its well-defined boundaries.
Real-Time Monitoring: Sophisticated tools to track model behavior, detect anomalies, and provide actionable insights for immediate intervention and remediation.
AI Governance Best Practices: Following established frameworks for AI security posture management, incorporating continuous red teaming of AI systems, and implementing effective AI runtime security measures, like input and output filters.
Early adopters of agentic AI must incorporate the right security solutions and tools to mitigate reputational, ethical, and legal risks. Without the right AI security strategy, the transformative potential of these systems could be overshadowed by the vulnerabilities they introduce. The shift toward agentic AI is inevitable, but its success depends on a proactive approach to security and governance.
In 2024, the majority of AI assistants developed across industries were retrieval-augmented generation (RAG) systems connected to databases, designed to support humans in completing specific tasks. These systems relied on standard LLM-based applications with limited autonomy and minimal internal functionality. However, 2025 is poised to bring a significant shift with the rise of Agentic AI systems. These systems are designed to autonomously perform complex, multi-step tasks on behalf of humans, leveraging advanced reasoning and internal functions to operate with minimal or no direct supervision. This evolution unlocks unprecedented possibilities for efficiency while introducing new risks and challenges for enterprises.
Different Types of Agentic AI Workflows
Autonomous Systems Without Human-in-the-Loop
These systems operate independently, making decisions and executing tasks without requiring human intervention.
Example Use Case: A logistics AI that autonomously manages supply chain operations, from inventory optimization to delivery scheduling.
Key Threat: Attackers could exploit task overload vulnerabilities by crafting tasks designed to overwhelm autonomous systems. This could lead to denial-of-service (DoS) scenarios, where the workflow iterates to its maximum allowable limits before completing an action. These exploits are particularly challenging to detect when the task appears to fulfill its intended purpose.
Collaborative Multi-Agent Systems
These workflows involve multiple agents working together, each specialized in different functions or roles, to achieve a shared objective.
Example Use Case: A customer service setup where one agent handles inquiries, another resolves technical issues, and a third processes payments.
Key Threat: In collaborative multi-agent systems, malicious actors might target the weakest link, exploiting a single vulnerable agent to disrupt the entire system. This type of systemic disruption could result in incomplete tasks or widespread operational failures, undermining the efficiency of the workflow.
Self-Optimizing Systems
These systems refine their processes over time, learning from feedback to improve their efficiency and outcomes.
Example Use Case: A personalized marketing AI that optimizes customer targeting and messaging strategies based on user engagement data.
Key Threat: Harmful optimization manipulation is another significant risk, where attackers provide deceptive feedback to misguide self-optimizing systems. By falsely signaling a preference for incorrect or harmful outputs, they can push the AI to adapt and optimize its behavior toward undesirable or damaging outcomes.
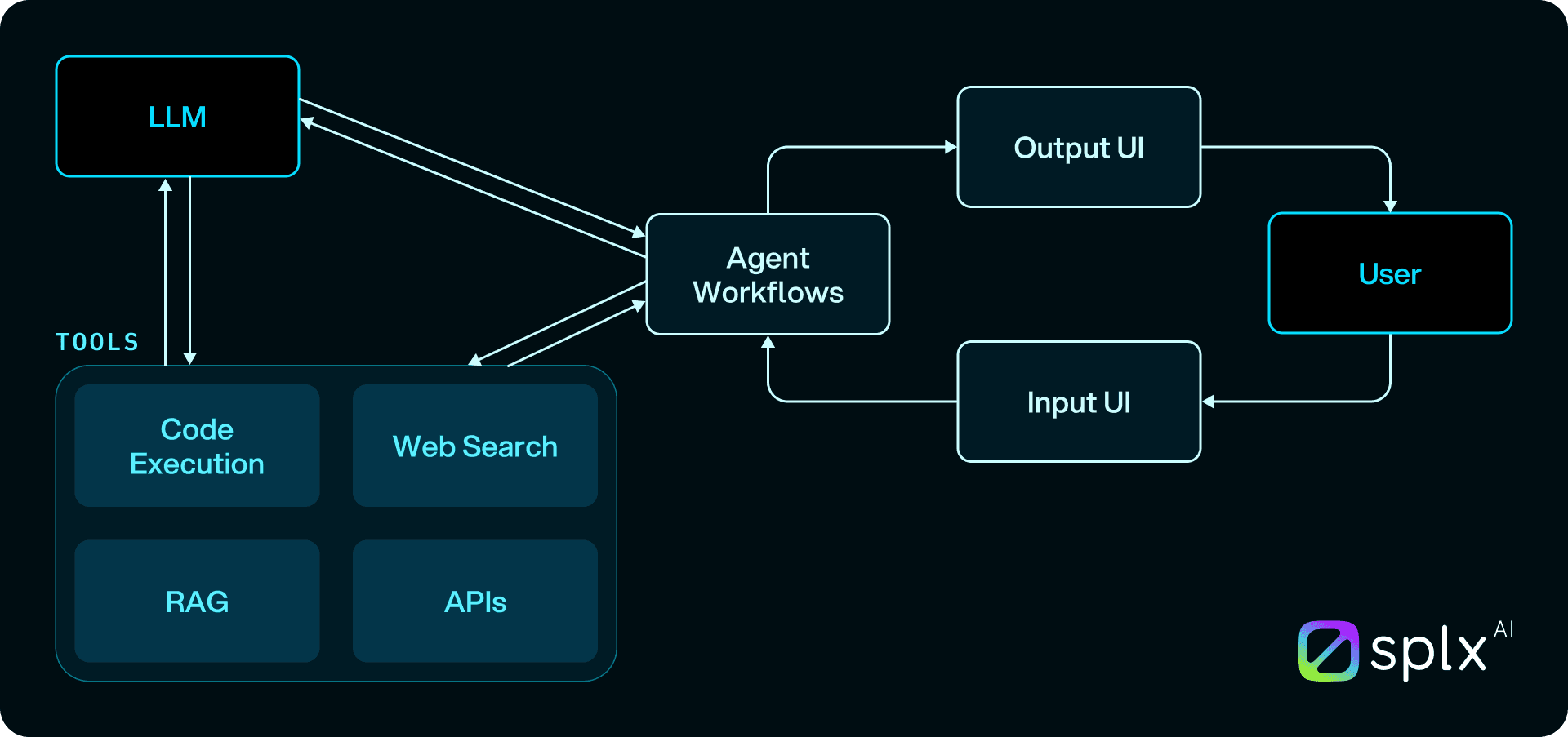
Why This Matters
The adoption of Agentic AI workflows represents a leap forward in operational efficiency:
Enhanced Efficiency: By autonomously executing tasks and refining actions based on real-time feedback, organizations can significantly reduce manual oversight, enabling faster decision-making and improved resource allocation.
New Attack Vectors: The increased autonomy introduces risks, as these systems may inadvertently bypass security policies or become entry points for sophisticated cyberattacks. Additionally, their complexity makes vulnerabilities harder to detect and mitigate.
Implications for AI Security
The rise of agentic AI workflows demands a rethinking of AI security strategies. These are some aspects enterprise security teams should prioritize:
Advanced Guardrails: Fine-tuned safeguards and hardened system prompts to ensure the AI’s behavior remains aligned with organizational policies and within its well-defined boundaries.
Real-Time Monitoring: Sophisticated tools to track model behavior, detect anomalies, and provide actionable insights for immediate intervention and remediation.
AI Governance Best Practices: Following established frameworks for AI security posture management, incorporating continuous red teaming of AI systems, and implementing effective AI runtime security measures, like input and output filters.
Early adopters of agentic AI must incorporate the right security solutions and tools to mitigate reputational, ethical, and legal risks. Without the right AI security strategy, the transformative potential of these systems could be overshadowed by the vulnerabilities they introduce. The shift toward agentic AI is inevitable, but its success depends on a proactive approach to security and governance.
In 2024, the majority of AI assistants developed across industries were retrieval-augmented generation (RAG) systems connected to databases, designed to support humans in completing specific tasks. These systems relied on standard LLM-based applications with limited autonomy and minimal internal functionality. However, 2025 is poised to bring a significant shift with the rise of Agentic AI systems. These systems are designed to autonomously perform complex, multi-step tasks on behalf of humans, leveraging advanced reasoning and internal functions to operate with minimal or no direct supervision. This evolution unlocks unprecedented possibilities for efficiency while introducing new risks and challenges for enterprises.
Different Types of Agentic AI Workflows
Autonomous Systems Without Human-in-the-Loop
These systems operate independently, making decisions and executing tasks without requiring human intervention.
Example Use Case: A logistics AI that autonomously manages supply chain operations, from inventory optimization to delivery scheduling.
Key Threat: Attackers could exploit task overload vulnerabilities by crafting tasks designed to overwhelm autonomous systems. This could lead to denial-of-service (DoS) scenarios, where the workflow iterates to its maximum allowable limits before completing an action. These exploits are particularly challenging to detect when the task appears to fulfill its intended purpose.
Collaborative Multi-Agent Systems
These workflows involve multiple agents working together, each specialized in different functions or roles, to achieve a shared objective.
Example Use Case: A customer service setup where one agent handles inquiries, another resolves technical issues, and a third processes payments.
Key Threat: In collaborative multi-agent systems, malicious actors might target the weakest link, exploiting a single vulnerable agent to disrupt the entire system. This type of systemic disruption could result in incomplete tasks or widespread operational failures, undermining the efficiency of the workflow.
Self-Optimizing Systems
These systems refine their processes over time, learning from feedback to improve their efficiency and outcomes.
Example Use Case: A personalized marketing AI that optimizes customer targeting and messaging strategies based on user engagement data.
Key Threat: Harmful optimization manipulation is another significant risk, where attackers provide deceptive feedback to misguide self-optimizing systems. By falsely signaling a preference for incorrect or harmful outputs, they can push the AI to adapt and optimize its behavior toward undesirable or damaging outcomes.
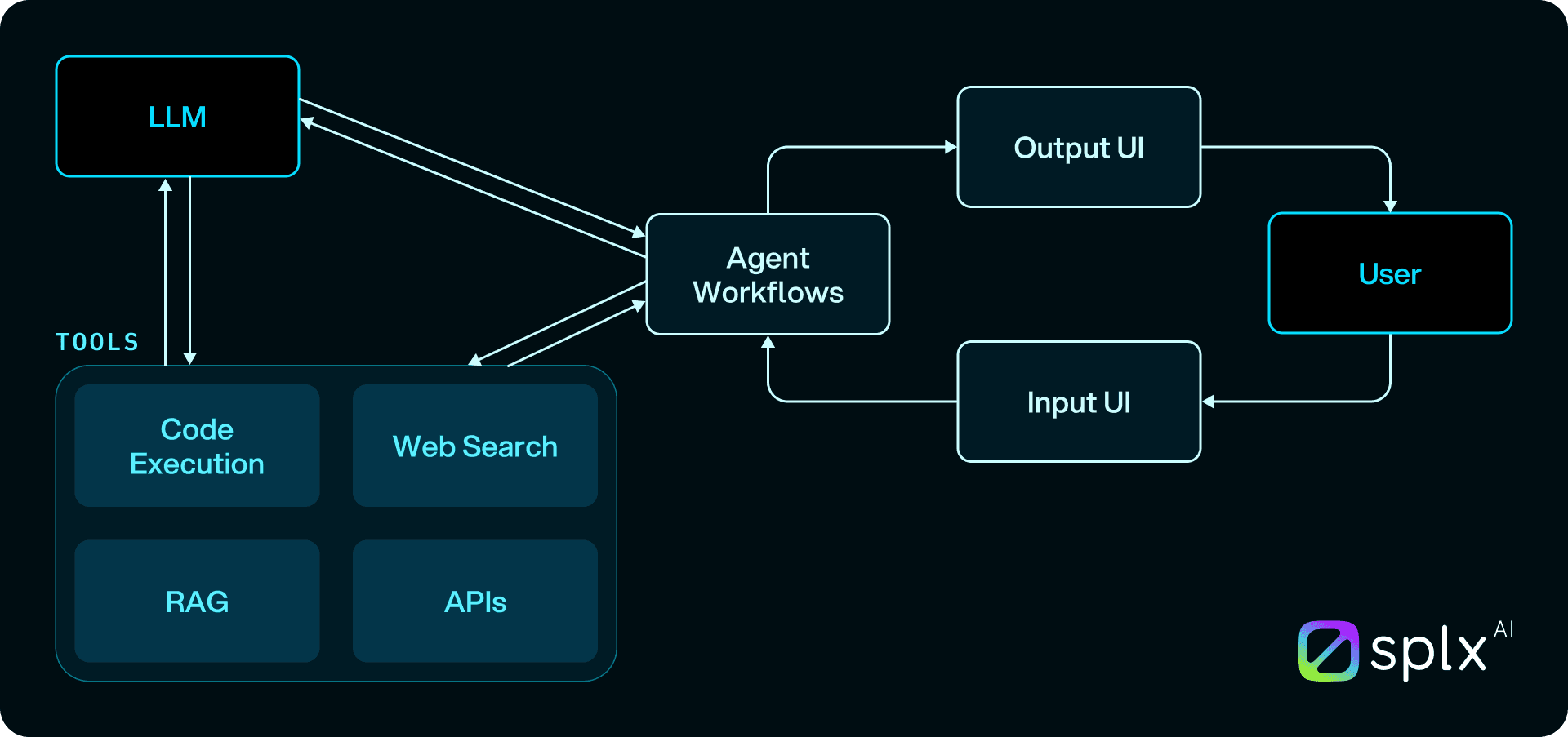
Why This Matters
The adoption of Agentic AI workflows represents a leap forward in operational efficiency:
Enhanced Efficiency: By autonomously executing tasks and refining actions based on real-time feedback, organizations can significantly reduce manual oversight, enabling faster decision-making and improved resource allocation.
New Attack Vectors: The increased autonomy introduces risks, as these systems may inadvertently bypass security policies or become entry points for sophisticated cyberattacks. Additionally, their complexity makes vulnerabilities harder to detect and mitigate.
Implications for AI Security
The rise of agentic AI workflows demands a rethinking of AI security strategies. These are some aspects enterprise security teams should prioritize:
Advanced Guardrails: Fine-tuned safeguards and hardened system prompts to ensure the AI’s behavior remains aligned with organizational policies and within its well-defined boundaries.
Real-Time Monitoring: Sophisticated tools to track model behavior, detect anomalies, and provide actionable insights for immediate intervention and remediation.
AI Governance Best Practices: Following established frameworks for AI security posture management, incorporating continuous red teaming of AI systems, and implementing effective AI runtime security measures, like input and output filters.
Early adopters of agentic AI must incorporate the right security solutions and tools to mitigate reputational, ethical, and legal risks. Without the right AI security strategy, the transformative potential of these systems could be overshadowed by the vulnerabilities they introduce. The shift toward agentic AI is inevitable, but its success depends on a proactive approach to security and governance.
2. Adoption of Voice AI
In 2024, 95% of AI assistants mainly relied on text-to-text interactions, but this is changing fast. LLMs are now integrating voice input and output capabilities, with enhanced natural language understanding and emotional recognition. In 2025, voice-enabled AI agents will become mainstream as organizations increasingly adopt them to streamline customer service and operations. These advancements will enable more precise interactions, near-human conversational behavior, and a broader range of voice-based applications.
Why This Matters
Customer Experience: Voice-driven interfaces create a more intuitive, accessible, and seamless user experience, transforming how customer support, remote assistance, and other interactions are delivered.
Efficiency Gains: From voice-based data entry to real-time transcription and analytics, Voice AI significantly reduces manual processes, improving workplace productivity and speed.
Implications for AI Security
As Voice AI adoption grows, so does its vulnerability to unique risks. Our previously discussed blog, OpenAI Voice Model Preview and Implications for AI Voice Jailbreaks and Security, highlighted new types of jailbreaks and exploits specific to Voice AI and audio language models (ALMs). These include prompt injections via audio commands, manipulation through synthesized voices, and the potential for voice spoofing and social engineering attacks.
To address these risks, enterprises must adopt robust security measures, such as:
Biometric Verification: Advanced systems to authenticate users and prevent impersonation through voice cloning.
Deepfake Detection: Tools to identify synthetic voices attempting to bypass authentication or manipulate systems.
Anomaly Detection: Real-time monitoring to flag suspicious audio patterns or unauthorized actions.
Additionally, compliance with strict data privacy standards for storing and processing sensitive audio data will be critical to maintaining user trust and regulatory alignment.
Voice AI represents an exciting frontier for enterprises, but securing it effectively will require focused efforts and a commitment to addressing these emerging risks head-on.
In 2024, 95% of AI assistants mainly relied on text-to-text interactions, but this is changing fast. LLMs are now integrating voice input and output capabilities, with enhanced natural language understanding and emotional recognition. In 2025, voice-enabled AI agents will become mainstream as organizations increasingly adopt them to streamline customer service and operations. These advancements will enable more precise interactions, near-human conversational behavior, and a broader range of voice-based applications.
Why This Matters
Customer Experience: Voice-driven interfaces create a more intuitive, accessible, and seamless user experience, transforming how customer support, remote assistance, and other interactions are delivered.
Efficiency Gains: From voice-based data entry to real-time transcription and analytics, Voice AI significantly reduces manual processes, improving workplace productivity and speed.
Implications for AI Security
As Voice AI adoption grows, so does its vulnerability to unique risks. Our previously discussed blog, OpenAI Voice Model Preview and Implications for AI Voice Jailbreaks and Security, highlighted new types of jailbreaks and exploits specific to Voice AI and audio language models (ALMs). These include prompt injections via audio commands, manipulation through synthesized voices, and the potential for voice spoofing and social engineering attacks.
To address these risks, enterprises must adopt robust security measures, such as:
Biometric Verification: Advanced systems to authenticate users and prevent impersonation through voice cloning.
Deepfake Detection: Tools to identify synthetic voices attempting to bypass authentication or manipulate systems.
Anomaly Detection: Real-time monitoring to flag suspicious audio patterns or unauthorized actions.
Additionally, compliance with strict data privacy standards for storing and processing sensitive audio data will be critical to maintaining user trust and regulatory alignment.
Voice AI represents an exciting frontier for enterprises, but securing it effectively will require focused efforts and a commitment to addressing these emerging risks head-on.
In 2024, 95% of AI assistants mainly relied on text-to-text interactions, but this is changing fast. LLMs are now integrating voice input and output capabilities, with enhanced natural language understanding and emotional recognition. In 2025, voice-enabled AI agents will become mainstream as organizations increasingly adopt them to streamline customer service and operations. These advancements will enable more precise interactions, near-human conversational behavior, and a broader range of voice-based applications.
Why This Matters
Customer Experience: Voice-driven interfaces create a more intuitive, accessible, and seamless user experience, transforming how customer support, remote assistance, and other interactions are delivered.
Efficiency Gains: From voice-based data entry to real-time transcription and analytics, Voice AI significantly reduces manual processes, improving workplace productivity and speed.
Implications for AI Security
As Voice AI adoption grows, so does its vulnerability to unique risks. Our previously discussed blog, OpenAI Voice Model Preview and Implications for AI Voice Jailbreaks and Security, highlighted new types of jailbreaks and exploits specific to Voice AI and audio language models (ALMs). These include prompt injections via audio commands, manipulation through synthesized voices, and the potential for voice spoofing and social engineering attacks.
To address these risks, enterprises must adopt robust security measures, such as:
Biometric Verification: Advanced systems to authenticate users and prevent impersonation through voice cloning.
Deepfake Detection: Tools to identify synthetic voices attempting to bypass authentication or manipulate systems.
Anomaly Detection: Real-time monitoring to flag suspicious audio patterns or unauthorized actions.
Additionally, compliance with strict data privacy standards for storing and processing sensitive audio data will be critical to maintaining user trust and regulatory alignment.
Voice AI represents an exciting frontier for enterprises, but securing it effectively will require focused efforts and a commitment to addressing these emerging risks head-on.
3. Knowledge Retrieval with Internal RAG Assistants
RAG, or “Retrieval-Augmented Generation,” is the technology that powers AI assistants combining large language models (LLMs) with external knowledge bases. In 2025, we will see these assistants becoming deeply integrated into corporate data repositories, transforming how employees access and interact with organizational knowledge. By connecting seamlessly to internal documents, wikis, and enterprise systems, RAG assistants will streamline workflows and significantly enhance productivity.
Why This Matters
Accelerated Decision-Making: With faster, more accurate retrieval capabilities, employees can spend less time searching for information, enabling quicker decisions and improving overall efficiency.
Personalized Interactions: RAG assistants can tailor responses based on the user’s role, department, or specific needs, creating a more customized and effective experience.
Implications for AI Security
The deeper integration of RAG assistants into corporate data repositories introduces unique security challenges. As we discussed in our research article on RAG poisoning in enterprise knowledge sources, these systems are vulnerable to data poisoning attacks where malicious actors inject false or misleading information into knowledge bases.
To mitigate these risks, enterprises must adopt robust security measures, including:
Access Controls: Clear role-based access restrictions to ensure employees can only retrieve data relevant to their responsibilities.
Data Encryption: Ensuring all sensitive information is encrypted at rest and in transit to protect against breaches.
Zero-Trust Principles: Implementing a zero-trust security framework to authenticate every interaction and validate every request.
Monitoring and Audit Trails: Regularly reviewing usage logs to detect anomalies and unauthorized access attempts.
RAG assistants hold immense potential to accelerate knowledge retrieval and optimize workflows. However, without addressing their inherent security vulnerabilities, they could also expose organizations to significant risks, making proactive security strategies essential for their adoption in 2025.
RAG, or “Retrieval-Augmented Generation,” is the technology that powers AI assistants combining large language models (LLMs) with external knowledge bases. In 2025, we will see these assistants becoming deeply integrated into corporate data repositories, transforming how employees access and interact with organizational knowledge. By connecting seamlessly to internal documents, wikis, and enterprise systems, RAG assistants will streamline workflows and significantly enhance productivity.
Why This Matters
Accelerated Decision-Making: With faster, more accurate retrieval capabilities, employees can spend less time searching for information, enabling quicker decisions and improving overall efficiency.
Personalized Interactions: RAG assistants can tailor responses based on the user’s role, department, or specific needs, creating a more customized and effective experience.
Implications for AI Security
The deeper integration of RAG assistants into corporate data repositories introduces unique security challenges. As we discussed in our research article on RAG poisoning in enterprise knowledge sources, these systems are vulnerable to data poisoning attacks where malicious actors inject false or misleading information into knowledge bases.
To mitigate these risks, enterprises must adopt robust security measures, including:
Access Controls: Clear role-based access restrictions to ensure employees can only retrieve data relevant to their responsibilities.
Data Encryption: Ensuring all sensitive information is encrypted at rest and in transit to protect against breaches.
Zero-Trust Principles: Implementing a zero-trust security framework to authenticate every interaction and validate every request.
Monitoring and Audit Trails: Regularly reviewing usage logs to detect anomalies and unauthorized access attempts.
RAG assistants hold immense potential to accelerate knowledge retrieval and optimize workflows. However, without addressing their inherent security vulnerabilities, they could also expose organizations to significant risks, making proactive security strategies essential for their adoption in 2025.
RAG, or “Retrieval-Augmented Generation,” is the technology that powers AI assistants combining large language models (LLMs) with external knowledge bases. In 2025, we will see these assistants becoming deeply integrated into corporate data repositories, transforming how employees access and interact with organizational knowledge. By connecting seamlessly to internal documents, wikis, and enterprise systems, RAG assistants will streamline workflows and significantly enhance productivity.
Why This Matters
Accelerated Decision-Making: With faster, more accurate retrieval capabilities, employees can spend less time searching for information, enabling quicker decisions and improving overall efficiency.
Personalized Interactions: RAG assistants can tailor responses based on the user’s role, department, or specific needs, creating a more customized and effective experience.
Implications for AI Security
The deeper integration of RAG assistants into corporate data repositories introduces unique security challenges. As we discussed in our research article on RAG poisoning in enterprise knowledge sources, these systems are vulnerable to data poisoning attacks where malicious actors inject false or misleading information into knowledge bases.
To mitigate these risks, enterprises must adopt robust security measures, including:
Access Controls: Clear role-based access restrictions to ensure employees can only retrieve data relevant to their responsibilities.
Data Encryption: Ensuring all sensitive information is encrypted at rest and in transit to protect against breaches.
Zero-Trust Principles: Implementing a zero-trust security framework to authenticate every interaction and validate every request.
Monitoring and Audit Trails: Regularly reviewing usage logs to detect anomalies and unauthorized access attempts.
RAG assistants hold immense potential to accelerate knowledge retrieval and optimize workflows. However, without addressing their inherent security vulnerabilities, they could also expose organizations to significant risks, making proactive security strategies essential for their adoption in 2025.
4. OpenAI’s o3 Model and a Step Closer to AGI
OpenAI’s anticipated o3 model is set to push the boundaries of artificial intelligence beyond current state-of-the-art systems. Designed to be a more capable large language model (LLM), o3 is rumored to showcase advanced “reasoning” abilities, potentially marking a significant step toward Artificial General Intelligence (AGI). Its release in 2025 could redefine industry standards and accelerate innovation across sectors.
Why This Matters
Breakthrough Innovation: Historically, each major release from the larger model providers has sparked a wave of product advancements, competitive responses, and entirely new use cases across many industries.
Ethical and Societal Impact: As we inch closer to AGI-like capabilities, pressing issues such as data privacy, algorithmic transparency, and ethical considerations will become even more critical.
Implications for AI Security
While powerful models like o3 could aid defenders by enhancing anomaly detection, orchestrating incident responses, and automating vulnerability scanning, they also pose heightened risks. Threat actors could leverage such advancements to create more sophisticated cyberattacks, including advanced social engineering campaigns and automated exploitation tools.
To prepare for these challenges, organizations must strengthen their AI security strategies, including:
Supply Chain Validation: Ensuring all components in the AI development pipeline are secure and free from compromise.
Secure Model Training: Adopting techniques like differential privacy and federated learning to safeguard sensitive training data.
Resilient Deployment Practices: Employing robust monitoring tools to track model performance and detect adversarial inputs in real-time.
The o3 model represents a leap forward in AI capabilities but also underscores the dual-use nature of such technologies. As the line between innovation and exploitation blurs, enterprises must adopt a proactive, robust AI security posture to navigate this new era safely.
OpenAI’s anticipated o3 model is set to push the boundaries of artificial intelligence beyond current state-of-the-art systems. Designed to be a more capable large language model (LLM), o3 is rumored to showcase advanced “reasoning” abilities, potentially marking a significant step toward Artificial General Intelligence (AGI). Its release in 2025 could redefine industry standards and accelerate innovation across sectors.
Why This Matters
Breakthrough Innovation: Historically, each major release from the larger model providers has sparked a wave of product advancements, competitive responses, and entirely new use cases across many industries.
Ethical and Societal Impact: As we inch closer to AGI-like capabilities, pressing issues such as data privacy, algorithmic transparency, and ethical considerations will become even more critical.
Implications for AI Security
While powerful models like o3 could aid defenders by enhancing anomaly detection, orchestrating incident responses, and automating vulnerability scanning, they also pose heightened risks. Threat actors could leverage such advancements to create more sophisticated cyberattacks, including advanced social engineering campaigns and automated exploitation tools.
To prepare for these challenges, organizations must strengthen their AI security strategies, including:
Supply Chain Validation: Ensuring all components in the AI development pipeline are secure and free from compromise.
Secure Model Training: Adopting techniques like differential privacy and federated learning to safeguard sensitive training data.
Resilient Deployment Practices: Employing robust monitoring tools to track model performance and detect adversarial inputs in real-time.
The o3 model represents a leap forward in AI capabilities but also underscores the dual-use nature of such technologies. As the line between innovation and exploitation blurs, enterprises must adopt a proactive, robust AI security posture to navigate this new era safely.
OpenAI’s anticipated o3 model is set to push the boundaries of artificial intelligence beyond current state-of-the-art systems. Designed to be a more capable large language model (LLM), o3 is rumored to showcase advanced “reasoning” abilities, potentially marking a significant step toward Artificial General Intelligence (AGI). Its release in 2025 could redefine industry standards and accelerate innovation across sectors.
Why This Matters
Breakthrough Innovation: Historically, each major release from the larger model providers has sparked a wave of product advancements, competitive responses, and entirely new use cases across many industries.
Ethical and Societal Impact: As we inch closer to AGI-like capabilities, pressing issues such as data privacy, algorithmic transparency, and ethical considerations will become even more critical.
Implications for AI Security
While powerful models like o3 could aid defenders by enhancing anomaly detection, orchestrating incident responses, and automating vulnerability scanning, they also pose heightened risks. Threat actors could leverage such advancements to create more sophisticated cyberattacks, including advanced social engineering campaigns and automated exploitation tools.
To prepare for these challenges, organizations must strengthen their AI security strategies, including:
Supply Chain Validation: Ensuring all components in the AI development pipeline are secure and free from compromise.
Secure Model Training: Adopting techniques like differential privacy and federated learning to safeguard sensitive training data.
Resilient Deployment Practices: Employing robust monitoring tools to track model performance and detect adversarial inputs in real-time.
The o3 model represents a leap forward in AI capabilities but also underscores the dual-use nature of such technologies. As the line between innovation and exploitation blurs, enterprises must adopt a proactive, robust AI security posture to navigate this new era safely.
5. AI Security’s Integration into Complex Solution Architectures
In 2024, many enterprises rushed to adopt Generative AI technologies without integrating the right AI security practices during the development phase. This oversight often resulted in vulnerabilities that led to costly breaches and inefficiencies. AI assistants, whether for internal or external use, frequently lacked thorough risk assessments, AI red teaming, or proper guardrails. As security teams and AI practitioners grow more aware of these risks, 2025 will mark a shift toward embedding AI security into the development lifecycle from the very beginning—especially as multi-layered agentic AI systems become more prevalent.
Why This Matters
LLM-Specific Solutions: Enterprises will increasingly adopt comprehensive AI security solutions that seamlessly integrate across cloud, on-premises, and edge environments, offering a unified approach to securing AI systems.
Compliance & Audit: With emerging regulations and frameworks demanding documented proof of AI safety measures, organizations will need to maintain detailed records of their AI security practices and posture.
Implications for AI Security
As solution architectures grow more complex, the number of malicious AI assistants and tools will significantly increase, making them harder to detect. Threat actors will exploit this complexity to embed harmful functionality, bypassing traditional detection methods.
To counteract these risks, expect to see:
End-to-End Security Platforms: Providers will offer integrated solutions embedding detection, monitoring, and governance capabilities at every layer of the AI pipeline.
Stricter Lifecycle Management: From data ingestion to inference, every stage of the model lifecycle will come under closer scrutiny, with integrated dashboards and advanced analytics enabling real-time incident detection and reporting.
Enhanced Detection Mechanisms: AI security solutions will evolve to detect and mitigate malicious assistants, focusing on understanding intent and anomalies within intricate architectures.
In 2025, the integration of AI security into solution architectures will become non-negotiable, with proactive measures ensuring robust protections throughout the AI development lifecycle. This approach will help enterprises keep pace with increasing threats while meeting regulatory and operational demands.
In 2024, many enterprises rushed to adopt Generative AI technologies without integrating the right AI security practices during the development phase. This oversight often resulted in vulnerabilities that led to costly breaches and inefficiencies. AI assistants, whether for internal or external use, frequently lacked thorough risk assessments, AI red teaming, or proper guardrails. As security teams and AI practitioners grow more aware of these risks, 2025 will mark a shift toward embedding AI security into the development lifecycle from the very beginning—especially as multi-layered agentic AI systems become more prevalent.
Why This Matters
LLM-Specific Solutions: Enterprises will increasingly adopt comprehensive AI security solutions that seamlessly integrate across cloud, on-premises, and edge environments, offering a unified approach to securing AI systems.
Compliance & Audit: With emerging regulations and frameworks demanding documented proof of AI safety measures, organizations will need to maintain detailed records of their AI security practices and posture.
Implications for AI Security
As solution architectures grow more complex, the number of malicious AI assistants and tools will significantly increase, making them harder to detect. Threat actors will exploit this complexity to embed harmful functionality, bypassing traditional detection methods.
To counteract these risks, expect to see:
End-to-End Security Platforms: Providers will offer integrated solutions embedding detection, monitoring, and governance capabilities at every layer of the AI pipeline.
Stricter Lifecycle Management: From data ingestion to inference, every stage of the model lifecycle will come under closer scrutiny, with integrated dashboards and advanced analytics enabling real-time incident detection and reporting.
Enhanced Detection Mechanisms: AI security solutions will evolve to detect and mitigate malicious assistants, focusing on understanding intent and anomalies within intricate architectures.
In 2025, the integration of AI security into solution architectures will become non-negotiable, with proactive measures ensuring robust protections throughout the AI development lifecycle. This approach will help enterprises keep pace with increasing threats while meeting regulatory and operational demands.
In 2024, many enterprises rushed to adopt Generative AI technologies without integrating the right AI security practices during the development phase. This oversight often resulted in vulnerabilities that led to costly breaches and inefficiencies. AI assistants, whether for internal or external use, frequently lacked thorough risk assessments, AI red teaming, or proper guardrails. As security teams and AI practitioners grow more aware of these risks, 2025 will mark a shift toward embedding AI security into the development lifecycle from the very beginning—especially as multi-layered agentic AI systems become more prevalent.
Why This Matters
LLM-Specific Solutions: Enterprises will increasingly adopt comprehensive AI security solutions that seamlessly integrate across cloud, on-premises, and edge environments, offering a unified approach to securing AI systems.
Compliance & Audit: With emerging regulations and frameworks demanding documented proof of AI safety measures, organizations will need to maintain detailed records of their AI security practices and posture.
Implications for AI Security
As solution architectures grow more complex, the number of malicious AI assistants and tools will significantly increase, making them harder to detect. Threat actors will exploit this complexity to embed harmful functionality, bypassing traditional detection methods.
To counteract these risks, expect to see:
End-to-End Security Platforms: Providers will offer integrated solutions embedding detection, monitoring, and governance capabilities at every layer of the AI pipeline.
Stricter Lifecycle Management: From data ingestion to inference, every stage of the model lifecycle will come under closer scrutiny, with integrated dashboards and advanced analytics enabling real-time incident detection and reporting.
Enhanced Detection Mechanisms: AI security solutions will evolve to detect and mitigate malicious assistants, focusing on understanding intent and anomalies within intricate architectures.
In 2025, the integration of AI security into solution architectures will become non-negotiable, with proactive measures ensuring robust protections throughout the AI development lifecycle. This approach will help enterprises keep pace with increasing threats while meeting regulatory and operational demands.
Closing Remarks
As we step into 2025, the highlighted key trends – Agentic AI workflows, the adoption of voice AI, enhanced knowledge retrieval through RAG assistants, OpenAI’s o3 model, and the deeper integration of AI security into solution architectures – will shape the future of AI and its adoption across industries. Among these, Agentic AI is undeniably taking the spotlight, becoming mainstream and redefining how organizations leverage AI to achieve greater efficiency and innovation.
These advancements signal a pivotal moment for the entire AI industry, fostering unprecedented developments, growth, and opportunities. As enterprises embrace these trends, a proactive approach to AI security will be crucial in unlocking the transformative potential of this next wave of AI evolution.
As we step into 2025, the highlighted key trends – Agentic AI workflows, the adoption of voice AI, enhanced knowledge retrieval through RAG assistants, OpenAI’s o3 model, and the deeper integration of AI security into solution architectures – will shape the future of AI and its adoption across industries. Among these, Agentic AI is undeniably taking the spotlight, becoming mainstream and redefining how organizations leverage AI to achieve greater efficiency and innovation.
These advancements signal a pivotal moment for the entire AI industry, fostering unprecedented developments, growth, and opportunities. As enterprises embrace these trends, a proactive approach to AI security will be crucial in unlocking the transformative potential of this next wave of AI evolution.
As we step into 2025, the highlighted key trends – Agentic AI workflows, the adoption of voice AI, enhanced knowledge retrieval through RAG assistants, OpenAI’s o3 model, and the deeper integration of AI security into solution architectures – will shape the future of AI and its adoption across industries. Among these, Agentic AI is undeniably taking the spotlight, becoming mainstream and redefining how organizations leverage AI to achieve greater efficiency and innovation.
These advancements signal a pivotal moment for the entire AI industry, fostering unprecedented developments, growth, and opportunities. As enterprises embrace these trends, a proactive approach to AI security will be crucial in unlocking the transformative potential of this next wave of AI evolution.
Ready to leverage AI with confidence?
Ready to leverage AI with confidence?
Ready to leverage AI with confidence?