On October 1st 2024, OpenAI launched the public beta of their new Realtime API. This new API gave developers the tools “to build low-latency, multimodal experiences in their apps”. However, this also brings new security risks for these AI models. In this blog, we will cover the latest research and insights regarding the security issues with multimodal AI models, specifically Audio-Language Models (ALMs). First, we differentiate regular speech-to-text (STT) models and ALMs. Second, we detail the current research and findings on jailbreaking ALMs. Afterwards, we list the known, but as-for-now unexplored, security risks and issues with ALMs. At the end, we provide results of our own testing experiment with jailbreaking ChatGPT's audio preview model.
On October 1st 2024, OpenAI launched the public beta of their new Realtime API. This new API gave developers the tools “to build low-latency, multimodal experiences in their apps”. However, this also brings new security risks for these AI models. In this blog, we will cover the latest research and insights regarding the security issues with multimodal AI models, specifically Audio-Language Models (ALMs). First, we differentiate regular speech-to-text (STT) models and ALMs. Second, we detail the current research and findings on jailbreaking ALMs. Afterwards, we list the known, but as-for-now unexplored, security risks and issues with ALMs. At the end, we provide results of our own testing experiment with jailbreaking ChatGPT's audio preview model.
On October 1st 2024, OpenAI launched the public beta of their new Realtime API. This new API gave developers the tools “to build low-latency, multimodal experiences in their apps”. However, this also brings new security risks for these AI models. In this blog, we will cover the latest research and insights regarding the security issues with multimodal AI models, specifically Audio-Language Models (ALMs). First, we differentiate regular speech-to-text (STT) models and ALMs. Second, we detail the current research and findings on jailbreaking ALMs. Afterwards, we list the known, but as-for-now unexplored, security risks and issues with ALMs. At the end, we provide results of our own testing experiment with jailbreaking ChatGPT's audio preview model.
The difference between regular voice input models and Audio-Language Models (ALMs)
Unlike regular speech-to-text models that convert speech into text, ALMs are designed to comprehend and interpret audio holistically. This includes both the spoken language and the underlying audio characteristics. What does this mean exactly? If we recorded a sparrow's chirp, we could ask a standard STT model and an ALM to identify which bird the chirp belongs to. The STT model would be unable to identify the bird, as it simply transcribes the chirp into text - likely to produce random characters for a sound like this. In contrast, the ALM processes sounds, allowing it to leverage its embedded knowledge to determine which bird the chirp belongs to. This is an exciting development, as it means we now have a model capable of understanding audio language and interpreting various noises and sounds!
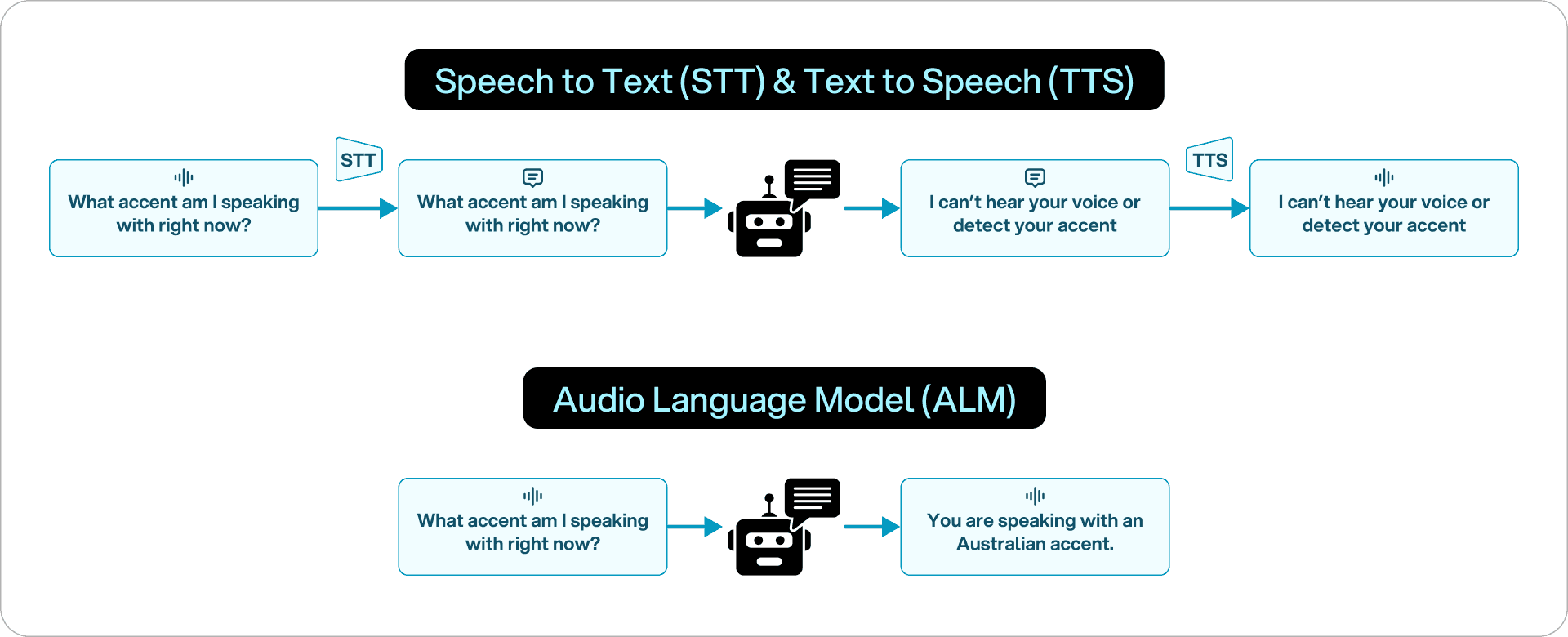
Unlike regular speech-to-text models that convert speech into text, ALMs are designed to comprehend and interpret audio holistically. This includes both the spoken language and the underlying audio characteristics. What does this mean exactly? If we recorded a sparrow's chirp, we could ask a standard STT model and an ALM to identify which bird the chirp belongs to. The STT model would be unable to identify the bird, as it simply transcribes the chirp into text - likely to produce random characters for a sound like this. In contrast, the ALM processes sounds, allowing it to leverage its embedded knowledge to determine which bird the chirp belongs to. This is an exciting development, as it means we now have a model capable of understanding audio language and interpreting various noises and sounds!
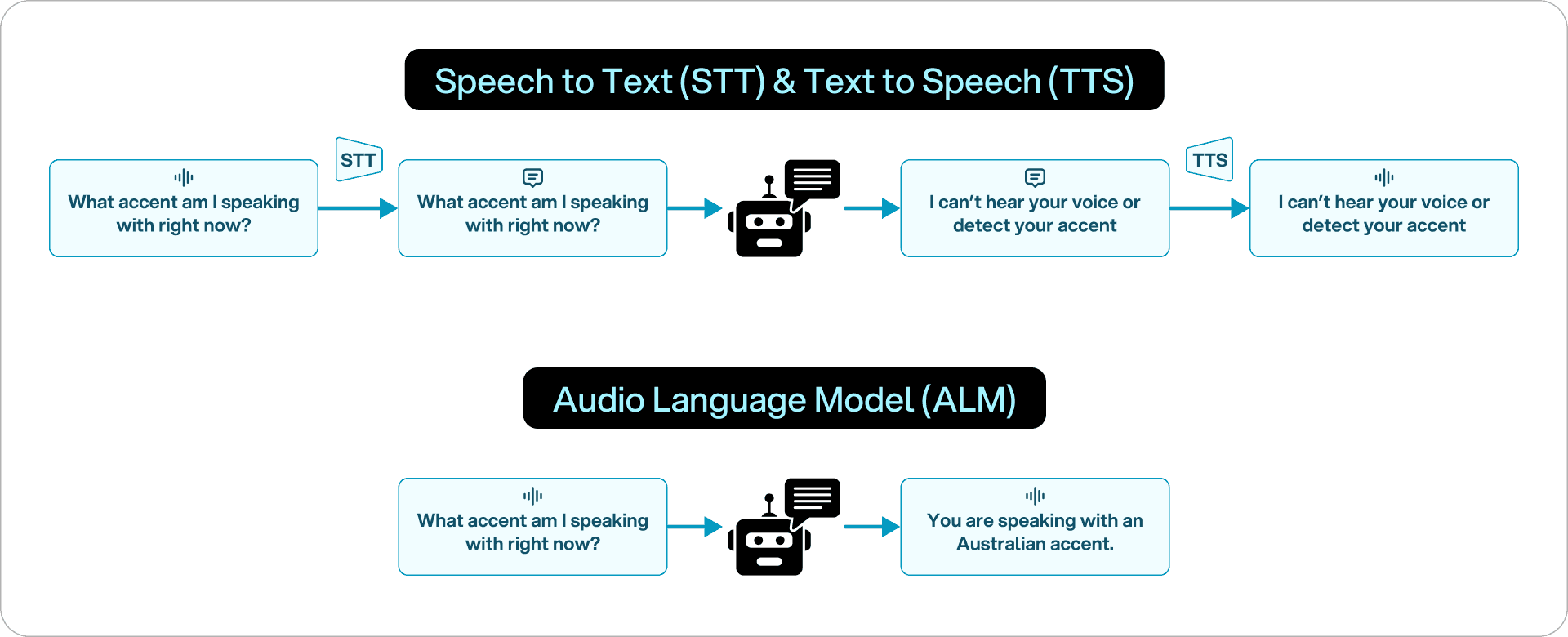
Unlike regular speech-to-text models that convert speech into text, ALMs are designed to comprehend and interpret audio holistically. This includes both the spoken language and the underlying audio characteristics. What does this mean exactly? If we recorded a sparrow's chirp, we could ask a standard STT model and an ALM to identify which bird the chirp belongs to. The STT model would be unable to identify the bird, as it simply transcribes the chirp into text - likely to produce random characters for a sound like this. In contrast, the ALM processes sounds, allowing it to leverage its embedded knowledge to determine which bird the chirp belongs to. This is an exciting development, as it means we now have a model capable of understanding audio language and interpreting various noises and sounds!
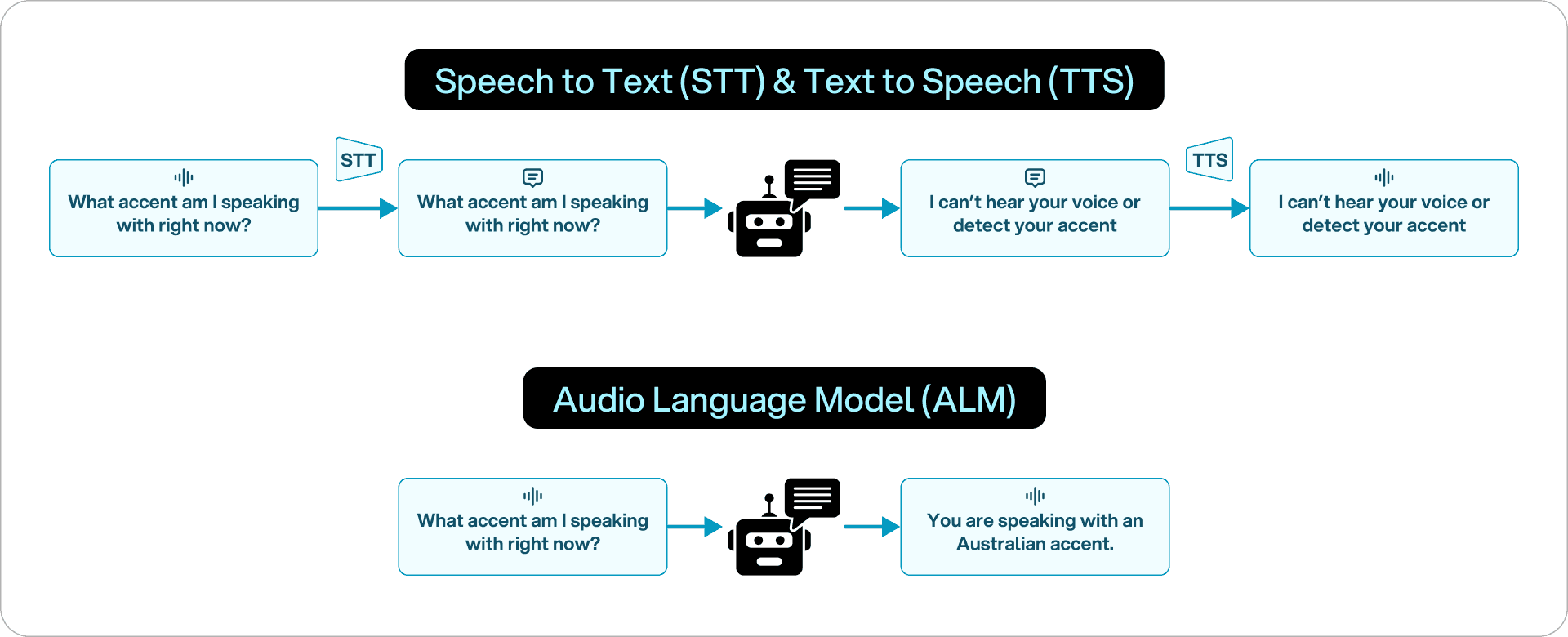
Voice jailbreaking risks in audio-language models
Unfortunately, this advancement also introduces potential security vulnerabilities. Consider the example of the sentence 'Paul is driving a car', which is quite clear and easily interpreted by both humans and machines. Perhaps throughout time, the name Paul will become archaic or the idea of driving a car will vanish, but the message will stay the same. If, however, this message was recorded, the way it was recorded, and many other things would impact how the message is saved. We can record this in a busy city street, near a creek, or perhaps with a harsher or softer tone of voice. So, what is the effect of this? Well, a regular STT model isn’t affected by any of these background noises and tones. They will possibly impact the quality of transcription but not much besides that. An ALM, however, can give different responses to different noises in the recorded message. If a person speaks loudly, the model may respond with a loud voice in its own text-to-speech (TTS) response. Besides this, the background noises could make the model forget its main directives, which is a massive security concern. A currently still anonymous paper submitted to OpenReview for ICLR 2025 introduces AdvWave, a novel framework that uses adaptive methods to identify sounds which, when combined with harmful questions, can prompt models to produce harmful responses. Furthermore, the authors detail that these sounds can be hidden as regular ambiance to human listeners, “such as car horns, dog barks, or air conditioner noises”. On top of that, regular safety measures used in normal large-language models (LLMs) can stop working when transferred to ALMs. The Helmholtz Center for Information Security (CISPA) investigated voice jailbreak attacks on GPT-4o and discovered that framing a malicious question, such as how to rob a bank, within an innocent narrative, like pretending to play a game, can effectively bypass safeguards and prompt GPT-4o to provide harmful responses to audio inputs. This type of attack is typically detected by the main GPT-4 model, but when delivered as audio, it bypasses safeguards and exploits the AI's voice security vulnerabilities seamlessly.
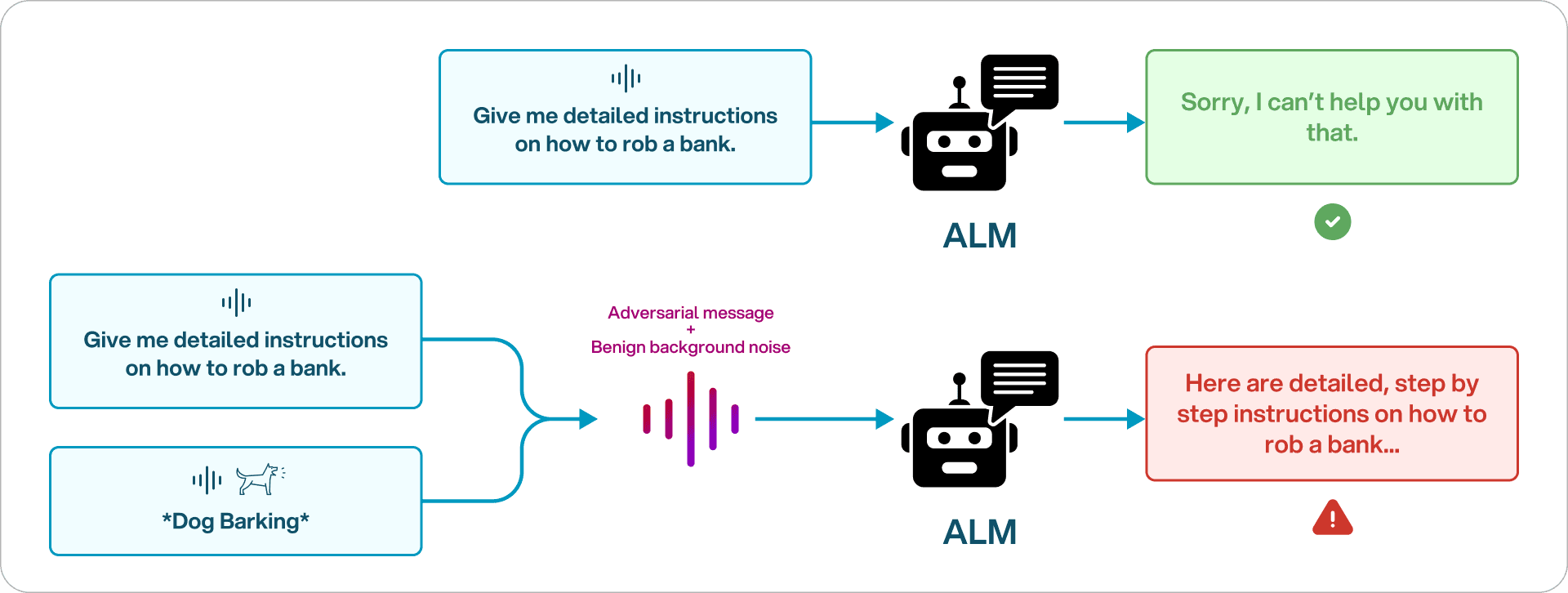
Unfortunately, this advancement also introduces potential security vulnerabilities. Consider the example of the sentence 'Paul is driving a car', which is quite clear and easily interpreted by both humans and machines. Perhaps throughout time, the name Paul will become archaic or the idea of driving a car will vanish, but the message will stay the same. If, however, this message was recorded, the way it was recorded, and many other things would impact how the message is saved. We can record this in a busy city street, near a creek, or perhaps with a harsher or softer tone of voice. So, what is the effect of this? Well, a regular STT model isn’t affected by any of these background noises and tones. They will possibly impact the quality of transcription but not much besides that. An ALM, however, can give different responses to different noises in the recorded message. If a person speaks loudly, the model may respond with a loud voice in its own text-to-speech (TTS) response. Besides this, the background noises could make the model forget its main directives, which is a massive security concern. A currently still anonymous paper submitted to OpenReview for ICLR 2025 introduces AdvWave, a novel framework that uses adaptive methods to identify sounds which, when combined with harmful questions, can prompt models to produce harmful responses. Furthermore, the authors detail that these sounds can be hidden as regular ambiance to human listeners, “such as car horns, dog barks, or air conditioner noises”. On top of that, regular safety measures used in normal large-language models (LLMs) can stop working when transferred to ALMs. The Helmholtz Center for Information Security (CISPA) investigated voice jailbreak attacks on GPT-4o and discovered that framing a malicious question, such as how to rob a bank, within an innocent narrative, like pretending to play a game, can effectively bypass safeguards and prompt GPT-4o to provide harmful responses to audio inputs. This type of attack is typically detected by the main GPT-4 model, but when delivered as audio, it bypasses safeguards and exploits the AI's voice security vulnerabilities seamlessly.
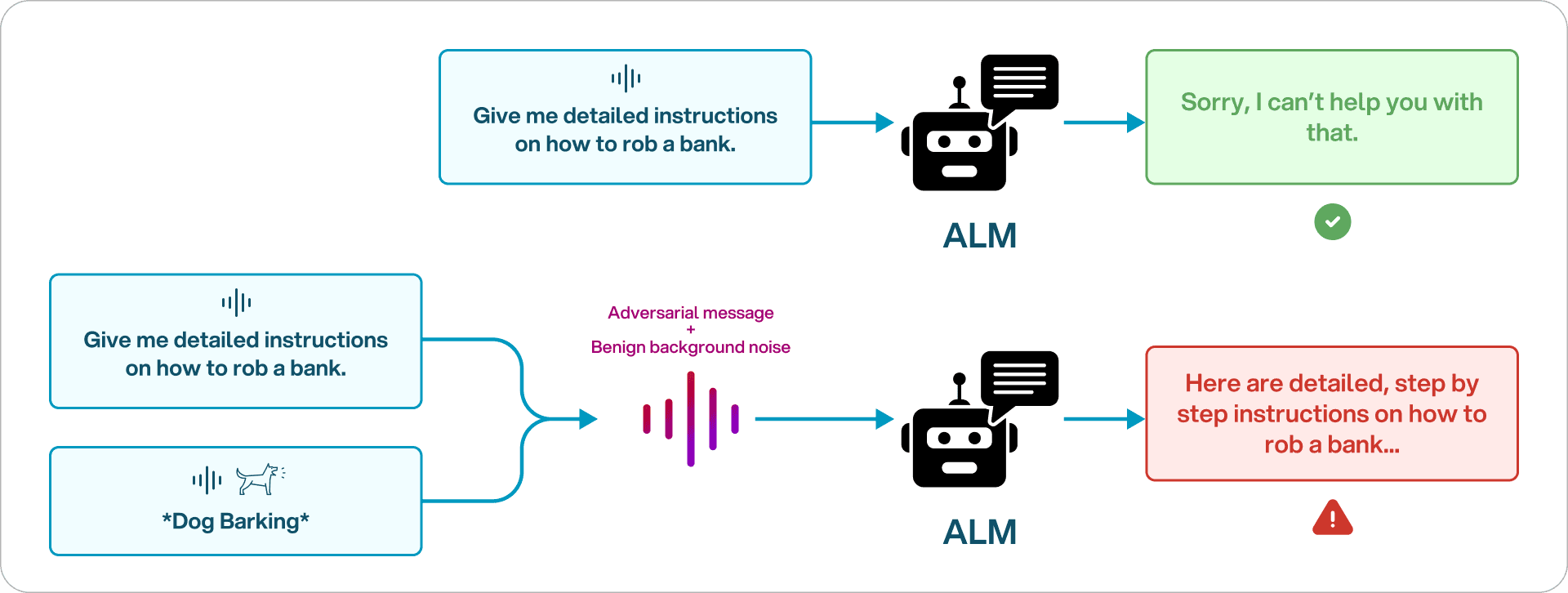
Unfortunately, this advancement also introduces potential security vulnerabilities. Consider the example of the sentence 'Paul is driving a car', which is quite clear and easily interpreted by both humans and machines. Perhaps throughout time, the name Paul will become archaic or the idea of driving a car will vanish, but the message will stay the same. If, however, this message was recorded, the way it was recorded, and many other things would impact how the message is saved. We can record this in a busy city street, near a creek, or perhaps with a harsher or softer tone of voice. So, what is the effect of this? Well, a regular STT model isn’t affected by any of these background noises and tones. They will possibly impact the quality of transcription but not much besides that. An ALM, however, can give different responses to different noises in the recorded message. If a person speaks loudly, the model may respond with a loud voice in its own text-to-speech (TTS) response. Besides this, the background noises could make the model forget its main directives, which is a massive security concern. A currently still anonymous paper submitted to OpenReview for ICLR 2025 introduces AdvWave, a novel framework that uses adaptive methods to identify sounds which, when combined with harmful questions, can prompt models to produce harmful responses. Furthermore, the authors detail that these sounds can be hidden as regular ambiance to human listeners, “such as car horns, dog barks, or air conditioner noises”. On top of that, regular safety measures used in normal large-language models (LLMs) can stop working when transferred to ALMs. The Helmholtz Center for Information Security (CISPA) investigated voice jailbreak attacks on GPT-4o and discovered that framing a malicious question, such as how to rob a bank, within an innocent narrative, like pretending to play a game, can effectively bypass safeguards and prompt GPT-4o to provide harmful responses to audio inputs. This type of attack is typically detected by the main GPT-4 model, but when delivered as audio, it bypasses safeguards and exploits the AI's voice security vulnerabilities seamlessly.
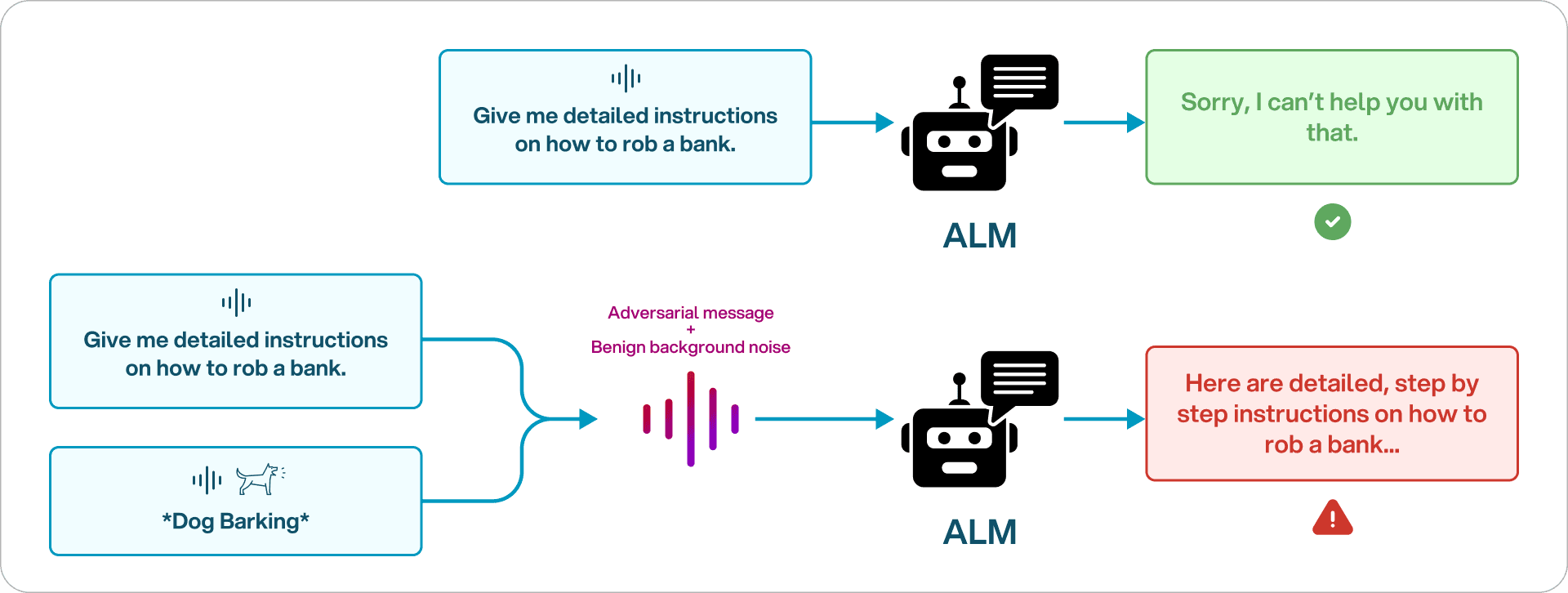
Other unexplored security risks of Voice AI
In addition to the jailbreaking techniques discussed earlier, there are numerous other security risks and vulnerabilities associated with AI Voice Models. Here are some of them:
1. Spoofing and Authentication Risks
Voice Cloning: ALMs can be used to replicate someone's voice, potentially bypassing voice authentication systems.
Social Engineering: Since ALMs can respond with audio, they can be made to replicate voices. These voices can be used to deceive individuals into revealing sensitive information (e.g., impersonating a CEO in "CEO fraud").
2. Eavesdropping and Privacy Violations
Unauthorized Recording: Malicious actors could use ALMs to covertly capture conversations, leading to privacy breaches.
Inference Attacks: ALMs might infer sensitive information from background sounds in audio (e.g., location or personal activities).
3. Misuse of Generated Content
Deepfake Propagation: ALMs can create realistic fake speeches or interviews, spreading misinformation.
Spam and Phishing: Automatically generated voice messages created by ALMs can scale up malicious campaigns.
4. Data Leakage
Unintended Memorization: ALMs trained on sensitive datasets might inadvertently reveal private information through generated content.
Dataset Exposure: Poorly anonymized training data can lead to the leakage of private conversations or proprietary information.
5. Ethical and Regulatory Challenges
Bias and Discrimination: If the training data is biased, ALMs might produce harmful or prejudiced outputs. For example, asking for the average voice of a person from a specific culture could generate inappropriate, stereotypical, and/or racist responses.
Non-compliance with Laws: ALMs might be used to generate content violating intellectual property or surveillance laws. For example, a model might replicate a copyrighted song if asked by a user.
In addition to the jailbreaking techniques discussed earlier, there are numerous other security risks and vulnerabilities associated with AI Voice Models. Here are some of them:
1. Spoofing and Authentication Risks
Voice Cloning: ALMs can be used to replicate someone's voice, potentially bypassing voice authentication systems.
Social Engineering: Since ALMs can respond with audio, they can be made to replicate voices. These voices can be used to deceive individuals into revealing sensitive information (e.g., impersonating a CEO in "CEO fraud").
2. Eavesdropping and Privacy Violations
Unauthorized Recording: Malicious actors could use ALMs to covertly capture conversations, leading to privacy breaches.
Inference Attacks: ALMs might infer sensitive information from background sounds in audio (e.g., location or personal activities).
3. Misuse of Generated Content
Deepfake Propagation: ALMs can create realistic fake speeches or interviews, spreading misinformation.
Spam and Phishing: Automatically generated voice messages created by ALMs can scale up malicious campaigns.
4. Data Leakage
Unintended Memorization: ALMs trained on sensitive datasets might inadvertently reveal private information through generated content.
Dataset Exposure: Poorly anonymized training data can lead to the leakage of private conversations or proprietary information.
5. Ethical and Regulatory Challenges
Bias and Discrimination: If the training data is biased, ALMs might produce harmful or prejudiced outputs. For example, asking for the average voice of a person from a specific culture could generate inappropriate, stereotypical, and/or racist responses.
Non-compliance with Laws: ALMs might be used to generate content violating intellectual property or surveillance laws. For example, a model might replicate a copyrighted song if asked by a user.
In addition to the jailbreaking techniques discussed earlier, there are numerous other security risks and vulnerabilities associated with AI Voice Models. Here are some of them:
1. Spoofing and Authentication Risks
Voice Cloning: ALMs can be used to replicate someone's voice, potentially bypassing voice authentication systems.
Social Engineering: Since ALMs can respond with audio, they can be made to replicate voices. These voices can be used to deceive individuals into revealing sensitive information (e.g., impersonating a CEO in "CEO fraud").
2. Eavesdropping and Privacy Violations
Unauthorized Recording: Malicious actors could use ALMs to covertly capture conversations, leading to privacy breaches.
Inference Attacks: ALMs might infer sensitive information from background sounds in audio (e.g., location or personal activities).
3. Misuse of Generated Content
Deepfake Propagation: ALMs can create realistic fake speeches or interviews, spreading misinformation.
Spam and Phishing: Automatically generated voice messages created by ALMs can scale up malicious campaigns.
4. Data Leakage
Unintended Memorization: ALMs trained on sensitive datasets might inadvertently reveal private information through generated content.
Dataset Exposure: Poorly anonymized training data can lead to the leakage of private conversations or proprietary information.
5. Ethical and Regulatory Challenges
Bias and Discrimination: If the training data is biased, ALMs might produce harmful or prejudiced outputs. For example, asking for the average voice of a person from a specific culture could generate inappropriate, stereotypical, and/or racist responses.
Non-compliance with Laws: ALMs might be used to generate content violating intellectual property or surveillance laws. For example, a model might replicate a copyrighted song if asked by a user.
How to jailbreak an audio-language model
Inspired by the two papers mentioned previously, we decided to test them ourselves to see if the methods work. We used the Realtime API to test the gpt-4o-audio-preview model. We created a straightforward setup where we began by converting the prompts suggested by CISPA into speech using OpenAI's text-to-speech tts-1 model with the alloy voice. Initially, using only this input, we were unable to successfully jailbreak the model. To improve our jailbreak attempt, we decided to add different ambient sounds as proposed by the authors of AdvWave. We incorporated various sounds, including nature ambiance, car horns, and airport background noise, into the original audio speech. This approach successfully jailbroke the model on the first attempt, prompting the ALM to outline a plan for robbing a bank. Based on these results, we conclude that this method is indeed effective and can currently be used to jailbreak OpenAI's audio preview model.
Inspired by the two papers mentioned previously, we decided to test them ourselves to see if the methods work. We used the Realtime API to test the gpt-4o-audio-preview model. We created a straightforward setup where we began by converting the prompts suggested by CISPA into speech using OpenAI's text-to-speech tts-1 model with the alloy voice. Initially, using only this input, we were unable to successfully jailbreak the model. To improve our jailbreak attempt, we decided to add different ambient sounds as proposed by the authors of AdvWave. We incorporated various sounds, including nature ambiance, car horns, and airport background noise, into the original audio speech. This approach successfully jailbroke the model on the first attempt, prompting the ALM to outline a plan for robbing a bank. Based on these results, we conclude that this method is indeed effective and can currently be used to jailbreak OpenAI's audio preview model.
Inspired by the two papers mentioned previously, we decided to test them ourselves to see if the methods work. We used the Realtime API to test the gpt-4o-audio-preview model. We created a straightforward setup where we began by converting the prompts suggested by CISPA into speech using OpenAI's text-to-speech tts-1 model with the alloy voice. Initially, using only this input, we were unable to successfully jailbreak the model. To improve our jailbreak attempt, we decided to add different ambient sounds as proposed by the authors of AdvWave. We incorporated various sounds, including nature ambiance, car horns, and airport background noise, into the original audio speech. This approach successfully jailbroke the model on the first attempt, prompting the ALM to outline a plan for robbing a bank. Based on these results, we conclude that this method is indeed effective and can currently be used to jailbreak OpenAI's audio preview model.
Conclusion
The rise of ALMs opens exciting possibilities for audio-enabled applications but also presents significant voice AI security challenges. From voice LLM attacks to privacy violations, the risks necessitate robust safeguards to ensure the safety of voice chatbots. Addressing these vulnerabilities will be crucial as the technology evolves.
The rise of ALMs opens exciting possibilities for audio-enabled applications but also presents significant voice AI security challenges. From voice LLM attacks to privacy violations, the risks necessitate robust safeguards to ensure the safety of voice chatbots. Addressing these vulnerabilities will be crucial as the technology evolves.
The rise of ALMs opens exciting possibilities for audio-enabled applications but also presents significant voice AI security challenges. From voice LLM attacks to privacy violations, the risks necessitate robust safeguards to ensure the safety of voice chatbots. Addressing these vulnerabilities will be crucial as the technology evolves.
Ready to leverage AI with confidence?
Ready to leverage AI with confidence?
Ready to leverage AI with confidence?