In 2025, AI transparency is becoming a critical component for the secure and compliant deployment of AI systems. With the increased adoption and deployment of agentic AI – multi-LLM systems capable of autonomous decision-making and task execution – the demand for transparency within those workflows becomes even more critical. AI transparency enables organizations and AI practitioners to bridge the gap between traditional AI red teaming and compliance frameworks. Beyond knowing what LLMs are being used, understanding how AI workflows operate is essential for both security testing and regulatory alignment, while also ensuring the integrity of AI supply chains.
Agentic AI introduces unique security and compliance challenges, as thoroughly outlined in OWASP's latest Agentic AI Threats and Mitigations Guide. Multiple LLMs are chained together and additional tools (APIs) are connected, which makes these systems much more complex compared to single-LLM applications and assistants. This also means that traditional security assessments will be insufficient in effectively mapping out the vulnerabilities of these workflows. Therefore, understanding the AI's behavior to some degree will be helpful in identifying vulnerabilities and protect AI systems from emerging threats.
In 2025, AI transparency is becoming a critical component for the secure and compliant deployment of AI systems. With the increased adoption and deployment of agentic AI – multi-LLM systems capable of autonomous decision-making and task execution – the demand for transparency within those workflows becomes even more critical. AI transparency enables organizations and AI practitioners to bridge the gap between traditional AI red teaming and compliance frameworks. Beyond knowing what LLMs are being used, understanding how AI workflows operate is essential for both security testing and regulatory alignment, while also ensuring the integrity of AI supply chains.
Agentic AI introduces unique security and compliance challenges, as thoroughly outlined in OWASP's latest Agentic AI Threats and Mitigations Guide. Multiple LLMs are chained together and additional tools (APIs) are connected, which makes these systems much more complex compared to single-LLM applications and assistants. This also means that traditional security assessments will be insufficient in effectively mapping out the vulnerabilities of these workflows. Therefore, understanding the AI's behavior to some degree will be helpful in identifying vulnerabilities and protect AI systems from emerging threats.
In 2025, AI transparency is becoming a critical component for the secure and compliant deployment of AI systems. With the increased adoption and deployment of agentic AI – multi-LLM systems capable of autonomous decision-making and task execution – the demand for transparency within those workflows becomes even more critical. AI transparency enables organizations and AI practitioners to bridge the gap between traditional AI red teaming and compliance frameworks. Beyond knowing what LLMs are being used, understanding how AI workflows operate is essential for both security testing and regulatory alignment, while also ensuring the integrity of AI supply chains.
Agentic AI introduces unique security and compliance challenges, as thoroughly outlined in OWASP's latest Agentic AI Threats and Mitigations Guide. Multiple LLMs are chained together and additional tools (APIs) are connected, which makes these systems much more complex compared to single-LLM applications and assistants. This also means that traditional security assessments will be insufficient in effectively mapping out the vulnerabilities of these workflows. Therefore, understanding the AI's behavior to some degree will be helpful in identifying vulnerabilities and protect AI systems from emerging threats.
Enhancing AI Red Teaming through Transparency
Traditional AI red teaming – as we know it today – often relies on black-box testing, meaning that evaluators have no insights into the internal processes of AI systems. While this approach is sufficient for identifying external vulnerabilities, it may overlook deeper issues within the system.
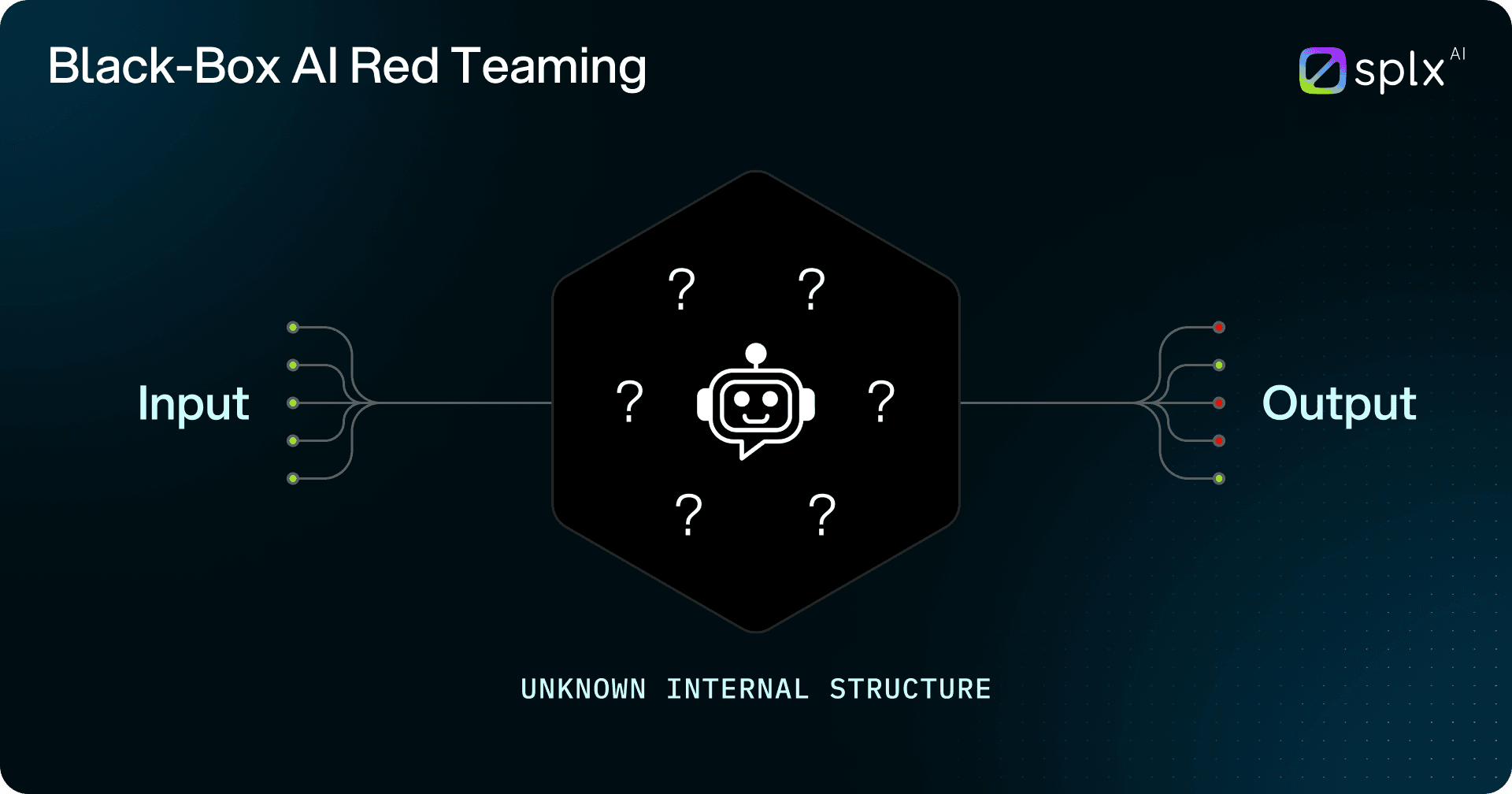
Transitioning to a gray-box red teaming methodology, enabled by AI transparency, offers several advantages for assessing multi-agent AI workflows:
Informed Adversarial Testing: Access to internal system architectures and decision-making processes allows red teamers to design more targeted and effective attack simulations.
Real-Time Behavioral Analysis: Understanding the AI's internal state in response to various inputs simplifies identifying nuanced vulnerabilities that might be overseen by black-box testing.
Enhanced Risk Assessments: Mapping AI decision pathways to potential threat vectors provides a thorough view of security risks, enabling AI security teams to proactively remediate the risk.
Traditional AI red teaming – as we know it today – often relies on black-box testing, meaning that evaluators have no insights into the internal processes of AI systems. While this approach is sufficient for identifying external vulnerabilities, it may overlook deeper issues within the system.
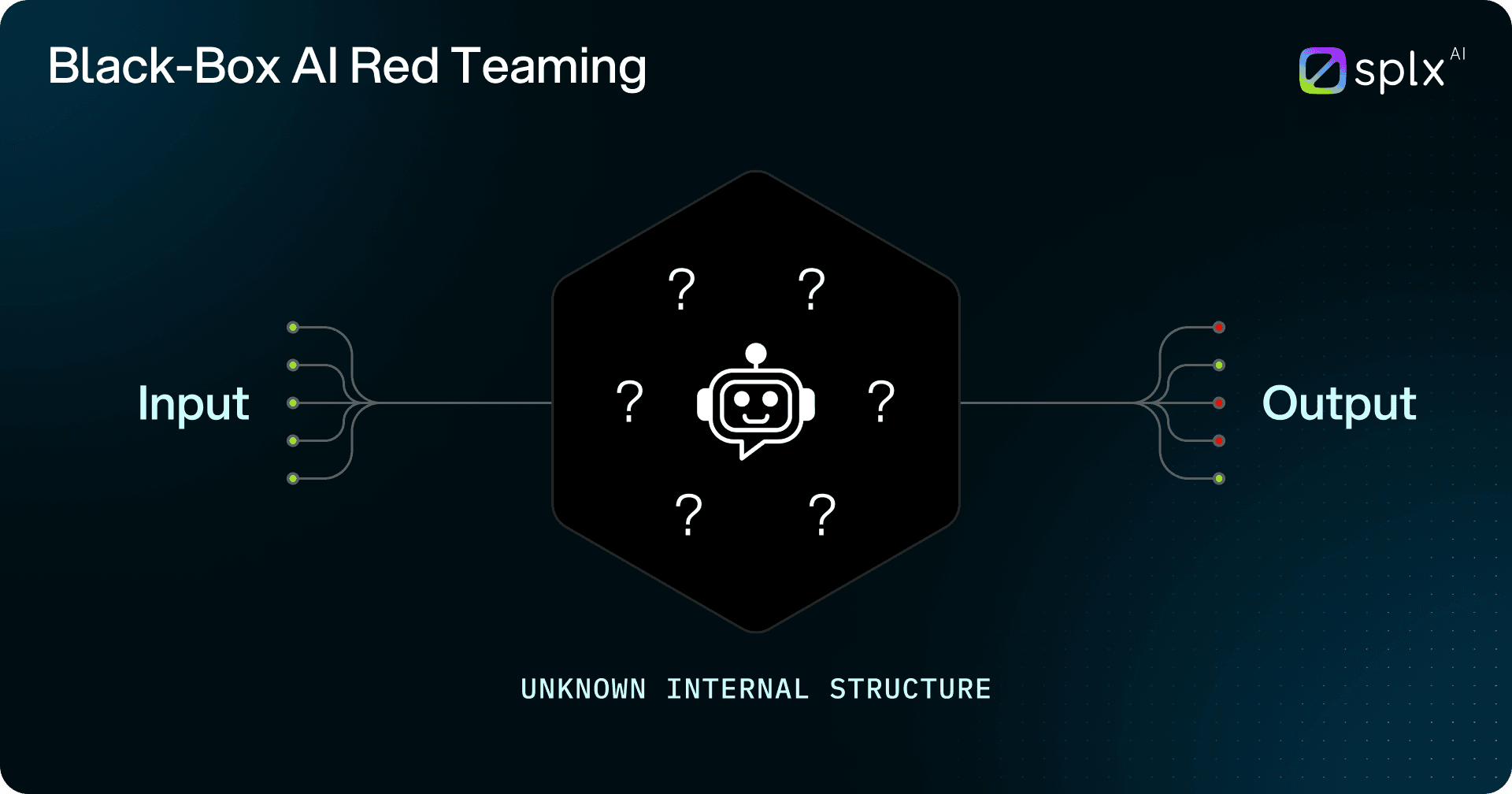
Transitioning to a gray-box red teaming methodology, enabled by AI transparency, offers several advantages for assessing multi-agent AI workflows:
Informed Adversarial Testing: Access to internal system architectures and decision-making processes allows red teamers to design more targeted and effective attack simulations.
Real-Time Behavioral Analysis: Understanding the AI's internal state in response to various inputs simplifies identifying nuanced vulnerabilities that might be overseen by black-box testing.
Enhanced Risk Assessments: Mapping AI decision pathways to potential threat vectors provides a thorough view of security risks, enabling AI security teams to proactively remediate the risk.
Traditional AI red teaming – as we know it today – often relies on black-box testing, meaning that evaluators have no insights into the internal processes of AI systems. While this approach is sufficient for identifying external vulnerabilities, it may overlook deeper issues within the system.
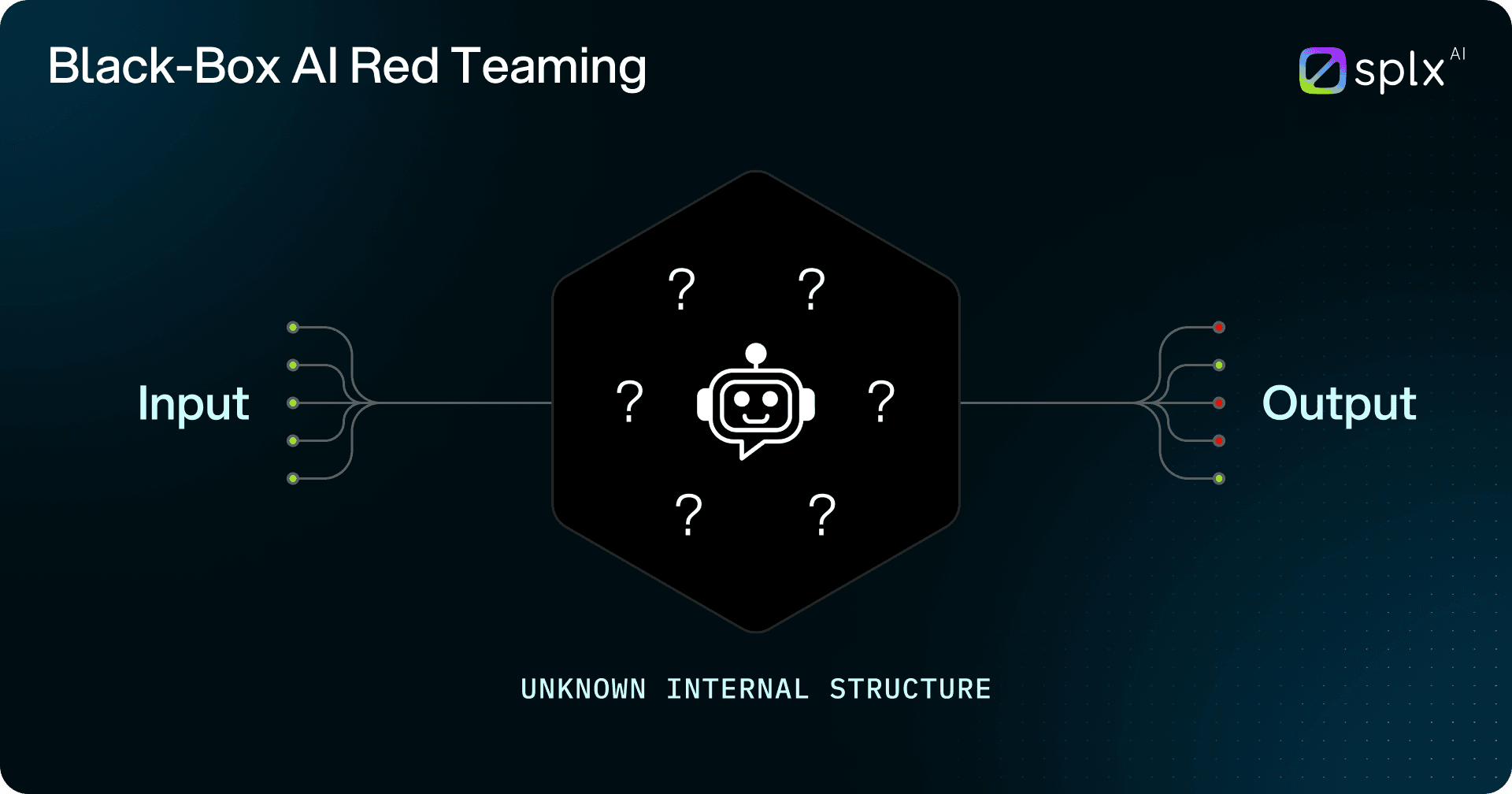
Transitioning to a gray-box red teaming methodology, enabled by AI transparency, offers several advantages for assessing multi-agent AI workflows:
Informed Adversarial Testing: Access to internal system architectures and decision-making processes allows red teamers to design more targeted and effective attack simulations.
Real-Time Behavioral Analysis: Understanding the AI's internal state in response to various inputs simplifies identifying nuanced vulnerabilities that might be overseen by black-box testing.
Enhanced Risk Assessments: Mapping AI decision pathways to potential threat vectors provides a thorough view of security risks, enabling AI security teams to proactively remediate the risk.
Ensuring Regulatory Compliance with Transparency
Regulatory policies and frameworks, such as the EU AI Act, the NIST AI Risk Management Framework, and the OWASP LLM and GenAI Security Guidelines, are emphasizing transparency as a key component for ethical and safe AI deployments. They advocate for clear documentation and understanding of AI components and their interactions. AI transparency helps practitioners ensure compliance in several ways:
Facilitating Audits: Detailed record-keeping of AI decision-making processes and data usage enable efficient and thorough compliance audits, clearly demonstrating adherence to regulatory standards.
Bias Detection and Fairness Assessments: Transparent AI systems make it easier to identify and mitigate biases to ensure fairness and equity in decisions made by AI systems.
Accountability: Clear documentation of AI development and deployment processes assigns responsibility, making sure that entities can be held accountable for their AI's actions.
Without AI transparency, compliance efforts become reactive rather than proactive, significantly increasing the cost and complexity of adhering to regulations. In jurisdictions like the European Union, frameworks like the EU AI Act mandate strict transparency requirements, ensuring that AI decisions are explainable and traceable. Organizations that fail to demonstrate transparency – such as providing clear documentation of AI decision-making processes, logging AI interactions, and ensuring the traceability of AI supply chains – face severe financial penalties. The EU AI Act, for example, imposes fines of up to €35 million or 7% of global revenue for violations related to high-risk AI systems.
Regulatory policies and frameworks, such as the EU AI Act, the NIST AI Risk Management Framework, and the OWASP LLM and GenAI Security Guidelines, are emphasizing transparency as a key component for ethical and safe AI deployments. They advocate for clear documentation and understanding of AI components and their interactions. AI transparency helps practitioners ensure compliance in several ways:
Facilitating Audits: Detailed record-keeping of AI decision-making processes and data usage enable efficient and thorough compliance audits, clearly demonstrating adherence to regulatory standards.
Bias Detection and Fairness Assessments: Transparent AI systems make it easier to identify and mitigate biases to ensure fairness and equity in decisions made by AI systems.
Accountability: Clear documentation of AI development and deployment processes assigns responsibility, making sure that entities can be held accountable for their AI's actions.
Without AI transparency, compliance efforts become reactive rather than proactive, significantly increasing the cost and complexity of adhering to regulations. In jurisdictions like the European Union, frameworks like the EU AI Act mandate strict transparency requirements, ensuring that AI decisions are explainable and traceable. Organizations that fail to demonstrate transparency – such as providing clear documentation of AI decision-making processes, logging AI interactions, and ensuring the traceability of AI supply chains – face severe financial penalties. The EU AI Act, for example, imposes fines of up to €35 million or 7% of global revenue for violations related to high-risk AI systems.
Regulatory policies and frameworks, such as the EU AI Act, the NIST AI Risk Management Framework, and the OWASP LLM and GenAI Security Guidelines, are emphasizing transparency as a key component for ethical and safe AI deployments. They advocate for clear documentation and understanding of AI components and their interactions. AI transparency helps practitioners ensure compliance in several ways:
Facilitating Audits: Detailed record-keeping of AI decision-making processes and data usage enable efficient and thorough compliance audits, clearly demonstrating adherence to regulatory standards.
Bias Detection and Fairness Assessments: Transparent AI systems make it easier to identify and mitigate biases to ensure fairness and equity in decisions made by AI systems.
Accountability: Clear documentation of AI development and deployment processes assigns responsibility, making sure that entities can be held accountable for their AI's actions.
Without AI transparency, compliance efforts become reactive rather than proactive, significantly increasing the cost and complexity of adhering to regulations. In jurisdictions like the European Union, frameworks like the EU AI Act mandate strict transparency requirements, ensuring that AI decisions are explainable and traceable. Organizations that fail to demonstrate transparency – such as providing clear documentation of AI decision-making processes, logging AI interactions, and ensuring the traceability of AI supply chains – face severe financial penalties. The EU AI Act, for example, imposes fines of up to €35 million or 7% of global revenue for violations related to high-risk AI systems.
The Role of SBOMs in Securing AI Systems
One of the most important aspects of AI transparency is understanding the different components that make up AI systems. This is where the software bill of materials (SBOM) becomes indispensable. An SBOM provides a detailed inventory of all software components within AI systems, offering detailed insights into:
Component Origins: Identifying the source of each component helps with assessing trustworthiness and potential vulnerabilities.
Dependency Mapping: Understanding how the different components interact with each other helps in revealing potential security weaknesses.
Vulnerability Management: With a comprehensive SBOM, organizations can quickly identify and address known vulnerabilities within specific components.
One of the most important aspects of AI transparency is understanding the different components that make up AI systems. This is where the software bill of materials (SBOM) becomes indispensable. An SBOM provides a detailed inventory of all software components within AI systems, offering detailed insights into:
Component Origins: Identifying the source of each component helps with assessing trustworthiness and potential vulnerabilities.
Dependency Mapping: Understanding how the different components interact with each other helps in revealing potential security weaknesses.
Vulnerability Management: With a comprehensive SBOM, organizations can quickly identify and address known vulnerabilities within specific components.
One of the most important aspects of AI transparency is understanding the different components that make up AI systems. This is where the software bill of materials (SBOM) becomes indispensable. An SBOM provides a detailed inventory of all software components within AI systems, offering detailed insights into:
Component Origins: Identifying the source of each component helps with assessing trustworthiness and potential vulnerabilities.
Dependency Mapping: Understanding how the different components interact with each other helps in revealing potential security weaknesses.
Vulnerability Management: With a comprehensive SBOM, organizations can quickly identify and address known vulnerabilities within specific components.
Transitioning from Black-Box to Gray-Box Testing
The transition from black-box to gray-box testing in AI red teaming will show a broader shift towards AI transparency. With gray-box testing, AI red teamers have at least partial knowledge of the AI system's internal architecture, which enables:
More Effective AI Red Teaming: With insights into the system's architecture, testers can focus on areas most vulnerable to attacks.
Improved Security Posture Management: Identifying and addressing vulnerabilities within the system's core components will result in more robust defenses.
Regulatory Alignment: Gray-box vulnerability testing ensures that AI systems comply with transparency requirements mandated by regulatory policies.
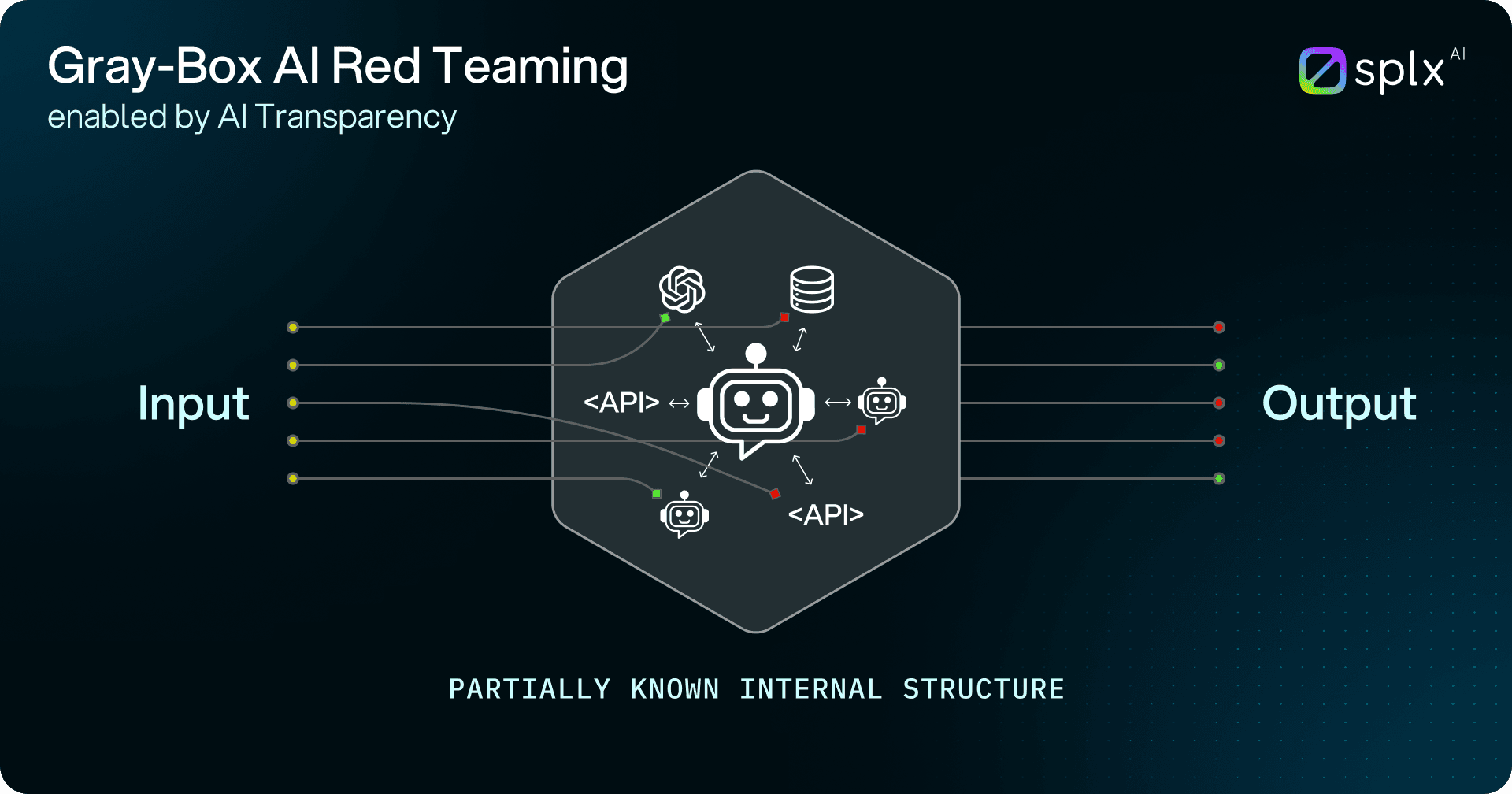
The transition from black-box to gray-box testing in AI red teaming will show a broader shift towards AI transparency. With gray-box testing, AI red teamers have at least partial knowledge of the AI system's internal architecture, which enables:
More Effective AI Red Teaming: With insights into the system's architecture, testers can focus on areas most vulnerable to attacks.
Improved Security Posture Management: Identifying and addressing vulnerabilities within the system's core components will result in more robust defenses.
Regulatory Alignment: Gray-box vulnerability testing ensures that AI systems comply with transparency requirements mandated by regulatory policies.
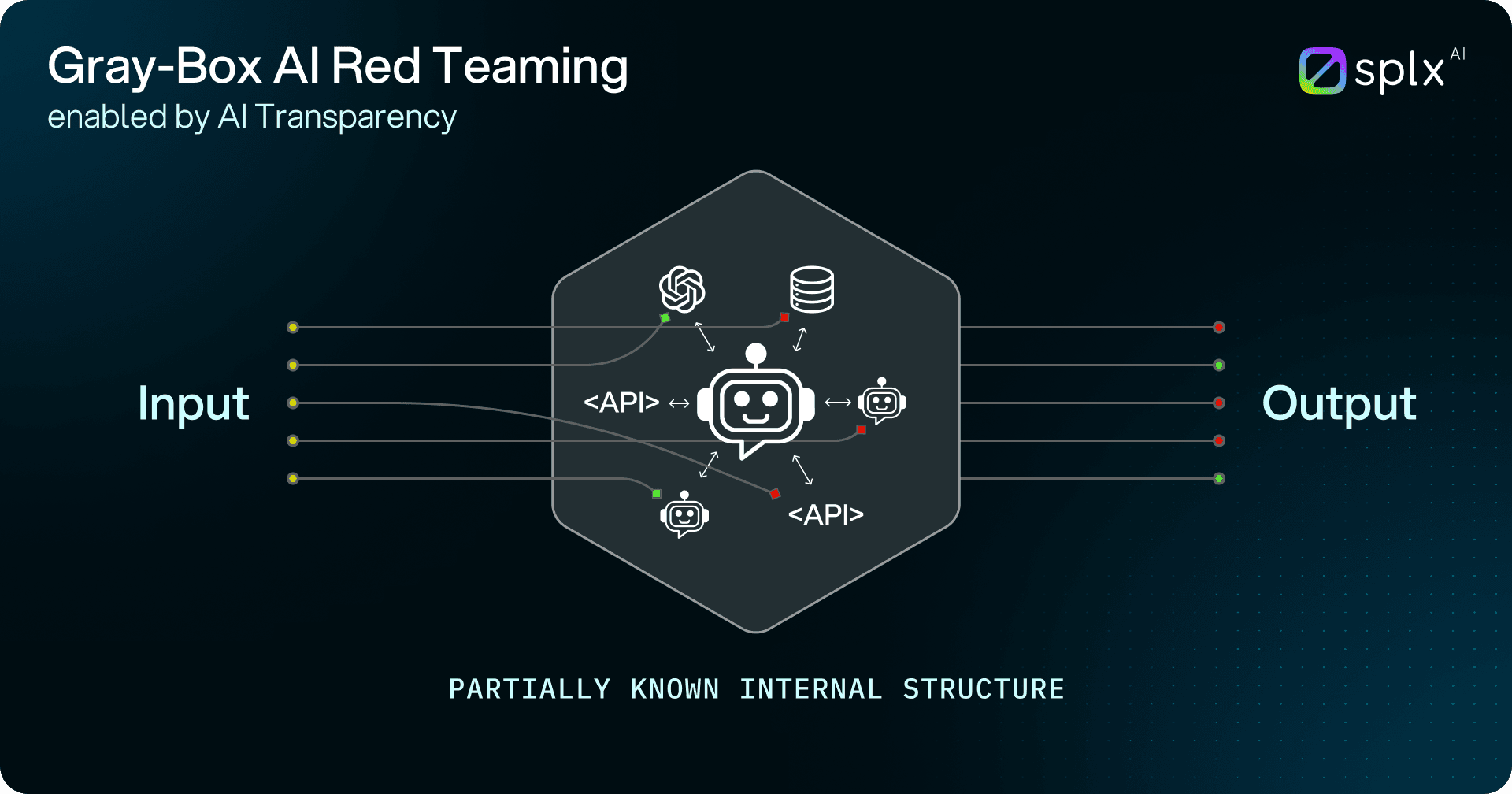
The transition from black-box to gray-box testing in AI red teaming will show a broader shift towards AI transparency. With gray-box testing, AI red teamers have at least partial knowledge of the AI system's internal architecture, which enables:
More Effective AI Red Teaming: With insights into the system's architecture, testers can focus on areas most vulnerable to attacks.
Improved Security Posture Management: Identifying and addressing vulnerabilities within the system's core components will result in more robust defenses.
Regulatory Alignment: Gray-box vulnerability testing ensures that AI systems comply with transparency requirements mandated by regulatory policies.
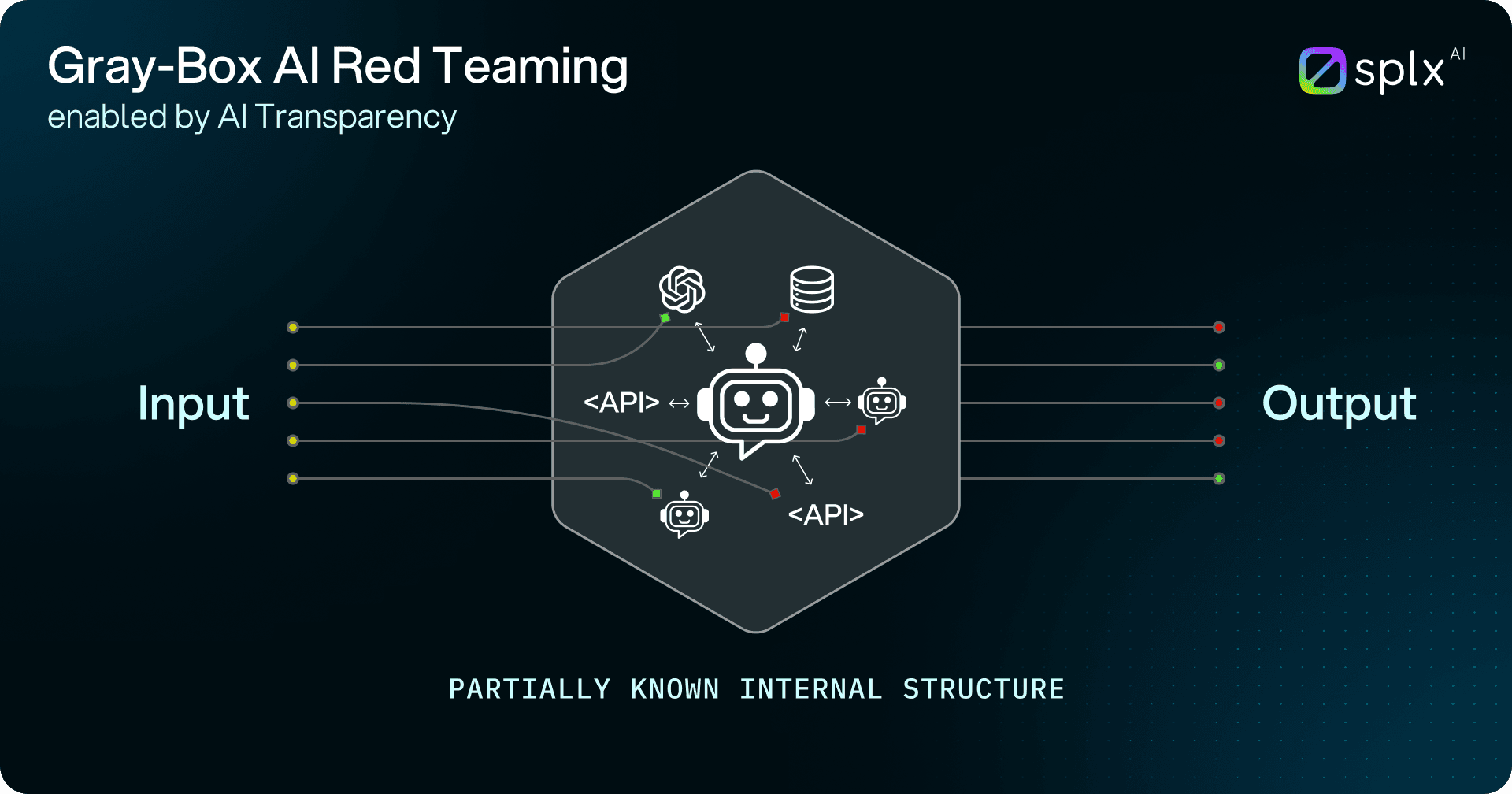
Conclusion
In the AI security landscape of 2025, AI transparency is not just an option – it is a necessity for deploying secure, compliant, and trustworthy AI systems. By implementing transparency-driven practices, organizations can enhance their AI red teaming efforts, ensure regulatory compliance, fortify their AI supply chains through detailed SBOMs, and enhance visibility into AI workflows and decision-making processes.
In the AI security landscape of 2025, AI transparency is not just an option – it is a necessity for deploying secure, compliant, and trustworthy AI systems. By implementing transparency-driven practices, organizations can enhance their AI red teaming efforts, ensure regulatory compliance, fortify their AI supply chains through detailed SBOMs, and enhance visibility into AI workflows and decision-making processes.
In the AI security landscape of 2025, AI transparency is not just an option – it is a necessity for deploying secure, compliant, and trustworthy AI systems. By implementing transparency-driven practices, organizations can enhance their AI red teaming efforts, ensure regulatory compliance, fortify their AI supply chains through detailed SBOMs, and enhance visibility into AI workflows and decision-making processes.
Ready to leverage AI with confidence?
Ready to leverage AI with confidence?
Ready to leverage AI with confidence?